Player FM - Internet Radio Done Right
19 subscribers
Checked 7d ago
Added four years ago
Content provided by Brian T. O’Neill from Designing for Analytics. All podcast content including episodes, graphics, and podcast descriptions are uploaded and provided directly by Brian T. O’Neill from Designing for Analytics or their podcast platform partner. If you believe someone is using your copyrighted work without your permission, you can follow the process outlined here https://player.fm/legal.
Player FM - Podcast App
Go offline with the Player FM app!
Go offline with the Player FM app!
Podcasts Worth a Listen
SPONSORED
V
Via Podcast


1 Close Encounters with UFO Hot Spots: Area 51, Roswell, and the Great ET Road Trip 39:50
39:50
Play Later
Play Later
Lists
Like
Liked39:50
The truth is out West! We’re hopping on the ET Highway and venturing to the most notorious alien hot spots, including Roswell’s infamous crash site, Area 51’s eerie perimeter, and a mysterious desert watchtower. Join us as journalist Laura Krantz, host of the podcast Wild Thing , beams up to share stories from the front lines of UFO reporting—from strange sightings and quirky festivals to a mailbox where people leave letters to extraterrestrials. Maybe you’ll even decide for yourself: Is Earth a tourist stop for spaceships? UFO hot spots you’ll encounter in this episode: - UFO Watchtower (near Great Sand Dunes National Park, Colorado) - Roswell, New Mexico - Area 51, Nevada - Extraterrestrial Highway (aka State Route 375), Nevada - Little A’Le’Inn, ET Highway, Nevada - E.T. Fresh Jerky, ET Highway, Nevada - Alien Research Center, ET Highway, Nevada - The Black Mailbox, ET Highway, Nevada Via Podcast is a production of AAA Mountain West Group .…
Experiencing Data w/ Brian T. O’Neill (UX for AI Data Products, SAAS Analytics, Data Product Management)
Mark all (un)played …
Manage series 2938687
Content provided by Brian T. O’Neill from Designing for Analytics. All podcast content including episodes, graphics, and podcast descriptions are uploaded and provided directly by Brian T. O’Neill from Designing for Analytics or their podcast platform partner. If you believe someone is using your copyrighted work without your permission, you can follow the process outlined here https://player.fm/legal.
Is the value of your enterprise analytics SAAS or AI product not obvious through it’s UI/UX? Got the data and ML models right...but user adoption of your dashboards and UI isn’t what you hoped it would be? While it is easier than ever to create AI and analytics solutions from a technology perspective, do you find as a founder or product leader that getting users to use and buyers to buy seems harder than it should be? If you lead an internal enterprise data team, have you heard that a ”data product” approach can help—but you’re concerned it’s all hype? My name is Brian T. O’Neill, and on Experiencing Data—one of the top 2% of podcasts in the world—I share the stories of leaders who are leveraging product and UX design to make SAAS analytics, AI applications, and internal data products indispensable to their customers. After all, you can’t create business value with data if the humans in the loop can’t or won’t use your solutions. Every 2 weeks, I release interviews with experts and impressive people I’ve met who are doing interesting work at the intersection of enterprise software product management, UX design, AI and analytics—work that you need to hear about and from whom I hope you can borrow strategies. I also occasionally record solo episodes on applying UI/UX design strategies to data products—so you and your team can unlock financial value by making your users’ and customers’ lives better. Hashtag: #ExperiencingData. JOIN MY INSIGHTS LIST FOR 1-PAGE EPISODE SUMMARIES, TRANSCRIPTS, AND FREE UX STRATEGY TIPS https://designingforanalytics.com/ed ABOUT THE HOST, BRIAN T. O’NEILL: https://designingforanalytics.com/bio/
…
continue reading
105 episodes
Mark all (un)played …
Manage series 2938687
Content provided by Brian T. O’Neill from Designing for Analytics. All podcast content including episodes, graphics, and podcast descriptions are uploaded and provided directly by Brian T. O’Neill from Designing for Analytics or their podcast platform partner. If you believe someone is using your copyrighted work without your permission, you can follow the process outlined here https://player.fm/legal.
Is the value of your enterprise analytics SAAS or AI product not obvious through it’s UI/UX? Got the data and ML models right...but user adoption of your dashboards and UI isn’t what you hoped it would be? While it is easier than ever to create AI and analytics solutions from a technology perspective, do you find as a founder or product leader that getting users to use and buyers to buy seems harder than it should be? If you lead an internal enterprise data team, have you heard that a ”data product” approach can help—but you’re concerned it’s all hype? My name is Brian T. O’Neill, and on Experiencing Data—one of the top 2% of podcasts in the world—I share the stories of leaders who are leveraging product and UX design to make SAAS analytics, AI applications, and internal data products indispensable to their customers. After all, you can’t create business value with data if the humans in the loop can’t or won’t use your solutions. Every 2 weeks, I release interviews with experts and impressive people I’ve met who are doing interesting work at the intersection of enterprise software product management, UX design, AI and analytics—work that you need to hear about and from whom I hope you can borrow strategies. I also occasionally record solo episodes on applying UI/UX design strategies to data products—so you and your team can unlock financial value by making your users’ and customers’ lives better. Hashtag: #ExperiencingData. JOIN MY INSIGHTS LIST FOR 1-PAGE EPISODE SUMMARIES, TRANSCRIPTS, AND FREE UX STRATEGY TIPS https://designingforanalytics.com/ed ABOUT THE HOST, BRIAN T. O’NEILL: https://designingforanalytics.com/bio/
…
continue reading
105 episodes
All episodes
×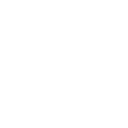
1 170 - Turning Data into Impactful AI Products at Experian: Lessons from North American Chief AI Officer Shri Santhnam (Promoted Episode) 42:33
42:33
Play Later
Play Later
Lists
Like
Liked42:33
Today, I'm chatting with Shri Santhanam, the EVP of Software Platforms and Chief AI Officer of Experian North America. Over the course of this promoted episode, you’re going to hear us talk about what it takes to build useful consumer and B2B AI products. Shri explains how Experian structures their AI product teams, the company’s approach prioritizing its initiatives, and what it takes to get their AI solutions out the door. We also get into the nuances of building trust with probabilistic AI tools and the absolutely critical role of UX in end user adoption. Note: today’s episode is one of my rare Promoted Episodes . Please help support the show by visiting Experian’s links below: Links Shri's LinkedIn Experian Assistant | Experian Experian Ascend Platform™ | Experian…
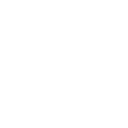
1 169 - AI Product Management and UX: What’s New (If Anything) About Making Valuable LLM-Powered Products with Stuart Winter-Tear 1:01:05
1:01:05
Play Later
Play Later
Lists
Like
Liked1:01:05
Today, I'm chatting with Stuart Winter-Tear about AI product management. We're getting into the nitty-gritty of what it takes to build and launch LLM-powered products for the commercial market that actually produce value. Among other things in this rich conversation, Stuart surprised me with the level of importance he believes UX has in making LLM-powered products successful, even for technical audiences. After spending significant time on the forefront of AI’s breakthroughs, Stuart believes many of the products we’re seeing today are the result of FOMO above all else. He shares a belief that I’ve emphasized time and time again on the podcast–product is about the problem, not the solution. This design philosophy has informed Staurt’s 20-plus year-long career, and it is pivotal to understanding how to best use AI to build products that meet users’ needs. Highlights/ Skip to Why Stuart was asked to speak to the House of Lords about AI (2:04) The LLM-powered products has Stuart been building recently (4:20) Finding product-market fit with AI products (7:44) Lessons Stuart has learned over the past two years working with LLM-power products (10:54) Figuring out how to build user trust in your AI products (14:40) The differences between being a digital product manager vs. AI product manager (18:13) Who is best suited for an AI product management role (25:42) Why Stuart thinks user experience matters greatly with AI products (32:18) The formula needed to create a business-viable AI product (38:22) Stuart describes the skills and roles he thinks are essential in an AI product team and who he brings on first (50:53) Conversations that need to be had with academics and data scientists when building AI-powered products (54:04) Final thoughts from Stuart and where you can find more from him (58:07) Quotes from Today’s Episode “I think that the core dream with GenAI is getting data out of IT hands and back to the business. Finding a way to overlay all this disparate, unstructured data and [translate it] to the human language is revolutionary. We’re finding industries that you would think were more conservative (i.e. medical, legal, etc.) are probably the most interested because of the large volumes of unstructured data they have to deal with. People wouldn’t expect large language models to be used for fact-checking… they’re actually very powerful, especially if you can have your own proprietary data or pipelines. Same with security–although large language models introduce a terrifying amount of security problems, they can also be used in reverse to augment security. There’s a lovely contradiction with this technology that I do enjoy.” - Stuart Winter-Tear (5:58) “[LLM-powered products] gave me the wow factor, and I think that’s part of what’s caused the problem. If we focus on technology, we build more technology, but if we focus on business and customers, we’re probably going to end up with more business and customers. This is why we end up with so many products that are effectively solutions in search of problems. We’re in this rush and [these products] are [based on] FOMO. We’re leaving behind what we understood about [building] products—as if [an LLM-powered product] is a special piece of technology. It’s not. It’s another piece of technology. [Designers] should look at this technology from the prism of the business and from the prism of the problem. We love to solutionize, but is the problem the problem? What’s the context of the problem? What’s the problem under the problem? Is this problem worth solving, and is GenAI a desirable way to solve it? We’re putting the cart before the horse.” - Stuart Winter-Tear (11:11) “[LLM-powered products] feel most amazing when you’re not a domain expert in whatever you’re using it for. I’ll give you an example: I’m terrible at coding. When I got my hands on Cursor, I felt like a superhero. It was unbelievable what I could build. Although [LLM products] look most amazing in the hands of non-experts, it’s actually most powerful in the hands of experts who do understand the domain they’re using this technology. Perhaps I want to do a product strategy, so I ask [the product] for some assistance, and it can get me 70% of the way there. [LLM products] are great as a jumping off point… but ultimately [they are] only powerful because I have certain domain expertise.” - Stuart Winter-Tear (13:01) “We’re so used to the digital paradigm. The deterministic nature of you put in X, you get out Y; it’s the same every time. Probabilistic changes every time. There is a huge difference between what results you might be getting in the lab compared to what happens in the real world. You effectively find yourself building [AI products] live, and in order to do that, you need good communities and good feedback available to you. You need these fast feedback loops. From a pure product management perspective, we used to just have the [engineering] timeline… Now, we have [the data research timeline]. If you’re dealing with cutting-edge products, you’ve got these two timelines that you’re trying to put together, and the data research one is very unpredictable. It’s the nature of research. We don’t necessarily know when we’re going to get to where we want to be.” - Stuart Winter-Tear (22:25) “I believe that UX will become the #1 priority for large language model products. I firmly believe whoever wins in UX will win in this large language model product world. I’m against fully autonomous agents without human intervention for knowledge work. We need that human in the loop. What was the intent of the user? How do we get that right push back from the large language model to understand even the level of the person that they’re dealing with? These are fundamental UX problems that are going to push UX to the forefront… This is going to be on UX to educate the user, to be able to inject the user in at the right time to be able to make this stuff work. The UX folk who do figure this out are going to create the breakthrough and create the mass adoption.” - Stuart Winter-Tear (33:42)…
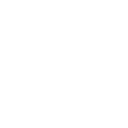
1 168 - 10 Challenges Internal Data Teams May Face Building Their First Revenue-Generating Data Product 38:24
38:24
Play Later
Play Later
Lists
Like
Liked38:24
Today, I am going to share some of the biggest challenges internal enterprise data leaders may face when creating their first revenue-generating data product. If your team is thinking about directly monetizing a data product and bringing a piece of software to life as something customers actually pay for, this episode is for you. As a companion to this episode, you can read my original article on this topic here once you finish listening!…
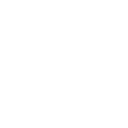
1 167 - AI Product Management and Design: How Natalia Andreyeva and Team at Infor Nexus Create B2B Data Products that Customers Value 37:34
37:34
Play Later
Play Later
Lists
Like
Liked37:34
Today, I’m talking with Natalia Andreyeva from Infor about AI / ML product management and its application to supply chain software. Natalia is a Senior Director of Product Management for the Nexus AI / ML Solution Portfolio, and she walks us through what is new, and what is not, about designing AI capabilities in B2B software. We also got into why user experience is so critical in data-driven products, and the role of design in ensuring AI produces value. During our chat, Natalia hit on the importance of really nailing down customer needs through solid discovery and the role of product leaders in this non-technical work. We also tackled some of the trickier aspects of designing for GenAI, digital assistants, the need to keep efforts strongly grounded in value creation for customers, and how even the best ML-based predictive analytics need to consider UX and the amount of evidence that customers need to believe the recommendations. During this episode, Natalia emphasizes a huge key to her work’s success: keeping customers and users in the loop throughout the product development lifecycle. Highlights/ Skip to What Natalia does as a Senior Director of Product Management for Infor Nexus (1:13) Who are the people using Infor Nexus Products and what do they accomplish when using them (2:51) Breaking down who makes up Natalia's team (4:05) What role does AI play in Natalia's work? (5:32) How do designers work with Natalia's team? (7:17) The problem that had Natalia rethink the discovery process when working with AI and machine learning applications (10:28) Why Natalia isn’t worried about competitors catching up to her team's design work (14:24) How Natalia works with Infor Nexus customers to help them understand the solutions her team is building (23:07) The biggest challenges Natalia faces with building GenAI and machine learning products (27:25) Natalia’s four steps to success in building AI products and capabilities (34:53) Where you can find more from Natalia (36:49) Quotes from Today’s Episode “I always launch discovery with customers, in the presence of the UX specialist [our designer]. We do the interviews together, and [regardless of who is facilitating] the goal is to understand the pain points of our customers by listening to how they do their jobs today. We do a series of these interviews and we distill them into the customer needs; the problems we need to really address for the customers. And then we start thinking about how to [address these needs]. Data products are a particular challenge because it’s not always that you can easily create a UX that would allow users to realize the value they’re searching for from the solution. And even if we can deliver it, consuming that is typically a challenge, too. So, this is where [design becomes really important]. [...] What I found through the years of experience is that it’s very difficult to explain to people around you what it is that you’re building when you’re dealing with a data-driven product. Is it a dashboard? Is it a workboard? They understand the word data, but that’s not what we are creating. We are creating the actual experience for the outcome that data will deliver to them indirectly, right? So, that’s typically how we work.” - Natalia Andreyeva (7:47) “[When doing discovery for products without AI], we already have ideas for what we want to get out. We know that there is a space in the market for those solutions to come to life. We just have to understand where. For AI-driven products, it’s not only about [the user’s] understanding of the problem or the design, it is also about understanding if the data exists and if it’s feasible to build the solution to address [the user’s] problem. [Data] feasibility is an extremely important piece because it will drive the UX as well.” - Natalia Andreyeva (10:50) “When [the team] discussed the problem, it sounded like a simple calculation that needed to be created [for users]. In reality, it was an entire process of thinking of multiple people in the chain [of command] to understand whether or not a medical product was safe to be consumed. That’s the outcome we needed to produce, and when we finally did, we actually celebrated with our customers and with our designers. It was one of the most difficult things that we had to design. So why did this problem actually get solved, and why we were the ones who solved it? It’s because we took the time to understand the current user experience through [our customer] interviews. We connected the dots and translated it all into a visual solution. We would never be able to do that without the proper UX and design in that place for the data.” - Natalia Andreyeva (13:16) “Everybody is pressured to come up with a strategy [for AI] or explain how AI is being incorporated into their solutions and platform, but it is still essential for all of my peers in product management to focus on the value [we’re] creating for customers. You cannot bypass discovery. Discovery is the essential portion where you have to spend time with your customers, champions, advisors, and their leads, but especially users who are doing this [supply chain] job every single day—so we understand where the pain point really is for them, we solve that pain, and we solve it with our design team as a partner, so that solution can surface value. ” - Natalia Andreyeva (22:08) “GenAI is a new field and new technology. It’s evolving quickly, and nobody really knows how to properly adapt or drive the adoption of AI solutions. The speed of innovation [in the AI field] is a challenge for everybody. People who work on the frontlines (i.e. product, engineering teams), have to stay way ahead of the market. Meanwhile, customers who are going to be using these [AI] solutions are not going to trust the [initial] outcomes. It’s going to take some time for people to become comfortable with them. But it doesn’t mean that your solution is bad or didn’t find the market fit. It’s just not time for your [solution] yet. Educating our users on the value of the solution is also part of that challenge, and [designers] have to be very careful that solutions are accessible. Users do not adopt intimidating solutions.” - Natalia Andreyeva (27:41) “First, discovery—where we search for the problems. From my experience, [discovery] works better if you’re very structured. I always provide [a customer] with an outline of what needs to happen so it’s not a secret. Then, do the prototyping phase and keep the customer engaged so they can see the quick outcomes of those prototypes. This is where you also have to really include the feasibility of the data if you’re building an AI solution, right? [Prototyping] can be short or long, but you need to keep the customer engaged throughout that phase so they see quick outcomes. Keep on validating this conceptually, you know, on the napkin, in Figma, it doesn’t really matter; you have to keep on keeping them engaged. Then, once you validate it works and the customer likes it, then build. Don’t really go into the deep development work until you know [all of this!] When you do build, create a beta solution. It only has to work so much to prove the value. Then, run the pilot, and if it’s successful, build the MVP, then launch. It’s simple, but it is a lot of work, and you have to keep your customers really engaged through all of those phases. If something doesn’t work [along the way], try to pivot early enough so you still have a viable product at the end.” - Natalia Andreyeva (34:53) Links Natalia's LinkedIn…
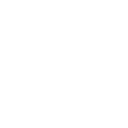
1 166 - Can UX Quality Metrics Increase Your Data Product's Business Value and Adoption? 26:12
26:12
Play Later
Play Later
Lists
Like
Liked26:12
Today I am going to try to answer a fundamental question: how should you actually measure user experience, especially with data products—and tie this to business value? It's easy to get lost in analytics and think we're seeing the whole picture, but I argue that this is far from the truth. Product leaders need to understand the subjective experience of our users—and unfortunately, analytics does not tell us this. The map is not the territory. In this episode, I discuss why qualitative data and subjective experience is the data that will most help you make product decisions that will lead you to increased business value. If users aren't getting value from your product(s), and their lives aren’t improving, business value will be extremely difficult to create. So today, I share my thoughts on how to move beyond thinking that analytics is the only way to track UX, and how this helps product leaders uncover opportunities to produce better organizational value. Ultimately, it’s about creating indispensable solutions and building trust, which is key for any product team looking to make a real impact. Hat tip to UX guru Jared Spool who inspired several of the concepts I share with you today. Highlights/ Skip to Don't target adoption for adoption's sake, because product usage can be a tax or benefit (3:00) Why your analytical mind may bias you—and what changes you might have to do this type of product and user research work (7:31) How "making the user's life better" translates to organizational value (10:17) Using Jared Spool's roller coaster chart to measure your product’s user experience and find your opportunities and successes (13:05) How do you measure that you have done a good job with your UX? (17:28) Conclusions and final thoughts (21:06) Quotes from Today’s Episode Usage doesn't automatically equal value. Analytics on your analytics is not telling you useful things about user experience or satisfaction. Why? "The map is not the territory." Analytics measure computer metrics, not feelings, and let's face it, users aren't always rational. To truly gauge user value, we need qualitative research - to talk to users - and to hear what their subjective experience is. Want *meaningful* adoption? Talk to and observe your users. That's how you know you are actually making things better. When it’s better for them, the business value will follow. (3:12) Make better things—where better is a measurement based on the subjective experience of the user—not analytics. Usable doesn’t mean they will necessarily want it. Sessions and page views don’t tell you how people *feel* about it. (7:39) Think about the dreadful tools you and so many have been forced to use: the things that waste your time and don’t let you focus on what’s really important. Ever talked to a data scientist who is sick of doing data prep instead of building models, and wondering, “why am I here? This isn’t what I went to school for.” Ignoring these personal frustrations and feelings and focusing only on your customers’ feature requests, JIRA tickets, stakeholder orders, requirements docs, and backlog items is why many teams end up building technically right, effectively wrong solutions. These end user frustrations are where we find our opportunities to delight—and create products and UXs that matter. To improve their lives, we need to dig into their workflows, identify frustrations, and understand the context around our data product solutions. Product leaders need to fall in love with the problems and the frustrations—these are the magic keys to the value kingdom. However, to do this well, you probably need to be doing less delivery and more discovery. (10:27) Imagine a line chart with a Y-axis that is "frustration" at the bottom to "delight" at the top. The X-axis is their user experience, taking place over time. As somebody uses your data product to do their job/task, you can plot their emotional journey. “Get the data, format the data, include the data in a tool, derive some conclusion, challenge the data, share it, make a decision” etc. As a product manager, you probably know what a use-case looks like. Your first job is to plot their existing experience trying/doing that use case with your data product. Where are they frustrated? Where are they delighted? Celebrate your peaks/delighters, and fall in love with the valleys where satisfaction work needs to be done. Connect the dots between these valleys and business value. Address the valleys—especially the ones that impede business value—and you’ll be on your way to “showing the value of your data product.” Analytics on your data product won’t tell you this information; the map is not the territory. (13:22) Analytics about your data product are lying to you. They give you the facts about the product, but not about the user. An example? “Time spent” doing a task. How long is too long? 5 minutes? 50? Analytics will tell you precisely how long it took. The problem is, it won’t tell you how long it FELT it took. And guess what? Your customers and users only care about how long it felt it took—vs. their expectation. Sure, at some point, analytics might eventually help—at scale—understand how your data product is doing—but first you have to understand how people FEEL about it. Only then will you know whether 5 minutes, or 50 minutes is telling you anything meaningful about what—if anything—needs to change. (16:17)…
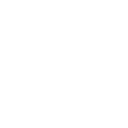
1 165 - How to Accommodate Multiple User Types and Needs in B2B Analytics and AI Products When You Lack UX Resources 49:04
49:04
Play Later
Play Later
Lists
Like
Liked49:04
A challenge I frequently hear about from subscribers to my insights mailing list is how to design B2B data products for multiple user types with differing needs. From dashboards to custom apps and commercial analytics / AI products, data product teams often struggle to create a single solution that meets the diverse needs of technical and business users in B2B settings. If you're encountering this issue, you're not alone! In this episode, I share my advice for tackling this challenge including the gift of saying "no.” What are the patterns you should be looking out for in your customer research? How can you choose what to focus on with limited resources? What are the design choices you should avoid when trying to build these products? I’m hoping by the end of this episode, you’ll have some strategies to help reduce the size of this challenge—particularly if you lack a dedicated UX team to help you sort through your various user/stakeholder demands. Highlights/ Skip to The importance of proper user research and clustering “jobs to be done” around business importance vs. task frequency—ignoring the rest until your solution can show measurable value (4:29) What “level” of skill to design for, and why “as simple as possible” isn’t what I generally recommend (13:44) When it may be advantageous to use role or feature-based permissions to hide/show/change certain aspects, UI elements, or features (19:50) Leveraging AI and LLMs in-product to allow learning about the user and progressive disclosure and customization of UIs (26:44) Leveraging the “old” solution of rapid prototyping—which is now faster than ever with AI, and can accelerate learning (capturing user feedback) (31:14) 5 things I do not recommend doing when trying to satisfy multiple user types in your b2b AI or analytics product (34:14) Quotes from Today’s Episode If you're not talking to your users and stakeholders sufficiently, you're going to have a really tough time building a successful data product for one user – let alone for multiple personas. Listen for repeating patterns in what your users are trying to achieve (tasks they are doing). Focus on the jobs and tasks they do most frequently or the ones that bring the most value to their business. Forget about the rest until you've proven that your solution delivers real value for those core needs. It's more about understanding the problems and needs, not just the solutions. The solutions tend to be easier to design when the problem space is well understood. Users often suggest solutions, but it's our job to focus on the core problem we're trying to solve; simply entering in any inbound requests verbatim into JIRA and then “eating away” at the list is not usually a reliable strategy. (5:52) I generally recommend not going for “easy as possible” at the cost of shallow value. Instead, you’re going to want to design for some “mid-level” ability, understanding that this may make early user experiences with the product more difficult. Why? Oversimplification can mislead because data is complex, problems are multivariate, and data isn't always ideal. There are also “n” number of “not-first” impressions users will have with your product. This also means there is only one “first impression” they have. As such, the idea conceptually is to design an amazing experience for the “n” experiences, but not to the point that users never realize value and give up on the product. While I'd prefer no friction, technical products sometimes will have to have a little friction up front however, don't use this as an excuse for poor design. This is hard to get right, even when you have design resources, and it’s why UX design matters as thinking this through ends up determining, in part, whether users obtain the promise of value you made to them. (14:21) As an alternative to rigid role and feature-based permissions in B2B data products, you might consider leveraging AI and / or LLMs in your UI as a means of simplifying and customizing the UI to particular users. This approach allows users to potentially interrogate the product about the UI, customize the UI, and even learn over time about the user’s questions (jobs to be done) such that becomes organically customized over time to their needs. This is in contrast to the rigid buckets that role and permission-based customization present. However, as discussed in my previous episode (164 - “The Hidden UX Taxes that AI and LLM Features Impose on B2B Customers Without Your Knowledge”) designing effective AI features and capabilities can also make things worse due to the probabilistic nature of the responses GenAI produces. As such, this approach may benefit from a UX designer or researcher familiar with designing data products. Understanding what “quality” means to the user, and how to measure it, is especially critical if you’re going to leverage AI and LLMs to make the product UX better. (20:13) The old solution of rapid prototyping is even more valuable now—because it’s possible to prototype even faster. However, prototyping is not just about learning if your solution is on track. Whether you use AI or pencil and paper, prototyping early in the product development process should be framed as a “prop to get users talking.” In other words, it is a prop to facilitate problem and need clarity—not solution clarity. Its purpose is to spark conversation and determine if you're solving the right problem. As you iterate, your need to continually validate the problem should shrink, which will present itself in the form of consistent feedback you hear from end users. This is the point where you know you can focus on the design of the solution. Innovation happens when we learn; so the goal is to increase your learning velocity. (31:35) Have you ever been caught in the trap of prioritizing feature requests based on volume? I get it. It's tempting to give the people what they think they want. For example, imagine ten users clamoring for control over specific parameters in your machine learning forecasting model. You could give them that control, thinking you're solving the problem because, hey, that's what they asked for! But did you stop to ask why they want that control? The reasons behind those requests could be wildly different. By simply handing over the keys to all the model parameters, you might be creating a whole new set of problems. Users now face a "usability tax," trying to figure out which parameters to lock and which to let float. The key takeaway? Focus on addressing the frequency that the same problems are occurring across your users, not just the frequency a given tactic or “solution” method (i.e. “model” or “dashboard” or “feature”) appears in a stakeholder or user request. Remember, problems are often disguised as solutions. We've got to dig deeper and uncover the real needs, not just address the symptoms. (36:19)…
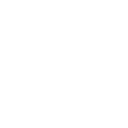
1 164 - The Hidden UX Taxes that AI and LLM Features Impose on B2B Customers Without Your Knowledge 45:25
45:25
Play Later
Play Later
Lists
Like
Liked45:25
Are you prepared for the hidden UX taxes that AI and LLM features might be imposing on your B2B customers—without your knowledge? Are you certain that your AI product or features are truly delivering value, or are there unseen taxes that are working against your users and your product / business? In this episode, I’m delving into some of UX challenges that I think need to be addressed when implementing LLM and AI features into B2B products. While AI seems to offer the change for significantly enhanced productivity, it also introduces a new layer of complexity for UX design. This complexity is not limited to the challenges of designing in a probabilistic medium (i.e. ML/AI), but also in being able to define what “quality” means. When the product team does not have a shared understanding of what a measurably better UX outcome means, improved sales and user adoption are less likely to follow. I’ll also discuss aspects of designing for AI that may be invisible on the surface. How might AI-powered products change the work of B2B users? What are some of the traps I see some startup clients and founders I advise in MIT’s Sandbox venture fund fall into? If you’re a product leader in B2B / enterprise software and want to make sure your AI capabilities don’t end up creating more damage than value for users, this episode will help! Highlights/ Skip to Improving your AI model accuracy improves outputs—but customers only care about outcomes (4:02) AI-driven productivity gains also put the customer’s “next problem” into their face sooner. Are you addressing the most urgent problem they now have—or used to have? (7:35) Products that win will combine AI with tastefully designed deterministic-software—because doing everything for everyone well is impossible and most models alone aren’t products (12:55) Just because your AI app or LLM feature can do ”X” doesn't mean people will want it or change their behavior (16:26) AI Agents sound great—but there is a human UX too, and it must enable trust and intervention at the right times (22:14) Not overheard from customers: “I would buy this/use this if it had AI” (26:52) Adaptive UIs sound like they’ll solve everything—but to reduce friction, they need to adapt to the person, not just the format of model outputs (30:20) Introducing AI introduces more states and scenarios that your product may need to support that may not be obvious right away (37:56) Quotes from Today’s Episode Product leaders have to decide how much effort and resources you should put into model improvements versus improving a user’s experience. Obviously, model quality is important in certain contexts and regulated industries, but when GenAI errors and confabulations are lower risk to the user (i.e. they create minor friction or inconveniences), the broader user experience that you facilitate might be what is actually determining the true value of your AI features or product. Model accuracy alone is not going to necessarily lead to happier users or increased adoption. ML models can be quantifiably tested for accuracy with structured tests, but because they’re easier to test for quality vs. something like UX doesn’t mean users value these improvements more. The product will stand a better chance of creating business value when it is clearly demonstrating it is improving your users’ lives. (5:25) When designing AI agents, there is still a human UX - a beneficiary - in the loop. They have an experience, whether you designed it with intention or not. How much transparency needs to be given to users when an agent does work for them? Should users be able to intervene when the AI is doing this type of work? Handling errors is something we do in all software, but what about retraining and learning so that the future user experiences is better? Is the system learning anything while it’s going through this—and can I tell if it’s learning what I want/need it to learn? What about humans in the loop who might interact with or be affected by the work the agent is doing even if they aren’t the agent’s owner or “user”? Who’s outcomes matter here? At what cost? (22:51) Customers primarily care about things like raising or changing their status, making more money, making their job easier, saving time, etc. In fact,I believe a product marketed with GenAI may eventually signal a negative / burden on customers thanks to the inflated and unmet expectations around AI that is poorly implemented in the product UX. Don’t think it’s going to be bought just because it using AI in a novel way. Customers aren’t sitting around wishing for “disruption” from your product; quite the opposite. AI or not, you need to make the customer the hero. Your AI will shine when it delivers an outsized UX outcome for your users (27:49) What kind of UX are you delivering right out of the box when a customer tries out your AI product or feature? Did you design it for tire kicking, playing around, and user stress testing? Or just an idealistic happy path? GenAI features inside b2b products should surface capabilities and constraints particularly around where users can create value for themselves quickly. Natural hints and well-designed prompt nudges in LLMs for example are important to users and to your product team: because you’re setting a more realistic expectation of what’s possible with customers and helping them get to an outcome sooner. You’re also teaching them how to use your solution to get the most value—without asking them to go read a manual. (38:21)…
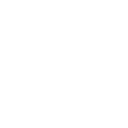
1 163 - It’s Not a Math Problem: How to Quantify the Value of Your Enterprise Data Products or Your Data Product Management Function 41:41
41:41
Play Later
Play Later
Lists
Like
Liked41:41
I keep hearing data product, data strategy, and UX teams often struggle to quantify the value of their work. Whether it’s as a team as a whole or on a specific data product initiative, the underlying problem is the same – your contribution is indirect, so it’s harder to measure. Even worse, your stakeholders want to know if your work is creating an impact and value, but because you can’t easily put numbers on it, valuation spirals into a messy problem. The messy part of this valuation problem is what today’s episode is all about—not math! Value is largely subjective, not objective, and I think this is partly why analytical teams may struggle with this. To improve at how you estimate the value of your data products, you need to leverage other skills—and stop approaching this as a math problem. As a consulting product designer, estimating value when it’s indirect is something that I’ve dealt with my entire career. It’s not a skill learned overnight, and it’s one you will need to keep developing over time—but the basic concepts are simple. I hope you’ll find some value in applying these along with your other frameworks and tools. Highlights/ Skip to Value is subjective, not objective (5:01) Measurability does not necessarily mean valuable (6:36) Businesses are made up of humans. Most b2b stakeholders aren’t spending their own money when making business decisions—what does that mean for your work? (9:30) Quantifying a data product’s value starts with understanding what is worth measuring in the eye of the beholder(s)—not math calculations (13:44) The more difficult it is to show the value of your product (or team) in numbers, the lower that value is to the stakeholder—initially (16:46) By simply helping a stakeholder to think through how value should be calculated on a data product, you’re likely already providing additional value (18:02) Focus on expressing estimated value via a range versus a single number (19:36) Measurement of anything requires that we can observe the phenomenon first—but many stakeholders won’t be able to cite these phenomena without [your!] help (22:16) When you are measuring quantitative aspects of value, remember that measurement is not the same as accuracy (precision)—and the precision game can become a trap (25:37) How to measure anything—and why estimates often trump accuracy (31:19) Why you may need to steer the conversation away from ROI calculations in the short term (35:00) Quotes from Today’s Episode Even when you can easily assign a dollar value to the data product you’re building, that does not necessarily reflect what your stakeholder actually feels about it—or your team’s contribution. So, why do they keep asking you to quantify the value of your work? By actually understanding what a shareholder needs to observe for them to know progress has been made on their initiative or data product, you will be positioned to deliver results they actually care about. While most of the time, you should be able to show some obvious economic value in the work you’re doing, you may be getting hounded about this because you’re not meeting the often unstated qualitative goals. If you can surface the qualitative goals of your stakeholder, then the perception of the value of your team and its work goes up, and you’ll spend less time trying to measure an indirect contribution in quant terms that only has a subjectively right answer. (6:50) The more difficult it is for you to show the monetary value of your data product (or team), the lower that value likely is to the stakeholder. This does not mean the value of your work is “low.” It means it’s perceived as low because it cannot be easily quantified in a way that is observable to the person whose judgment matters. By understanding the personal motivations and interests of your stakeholders, you can begin to collaboratively figure out what the correct success metrics should be—and how they’d be measured. By just simply beginning to ask and uncover what they’re trying to measure, you can start to increase your contributions’ perceived value. (17:01) Think about expressing “indirect value” as a range, not a precise single value. It’s much easier to refine your estimate (if necessary) once a range has been defined, and you only need to get precise enough for your stakeholder to make a decision with the information. How much time should you spend refining your measurement of the value? Potentially little to none—if the “better math” isn’t going to change anyone’s mind or decision. Spending more time to measure a data product’s value more accurately takes you away from doing actual product work—and if there isn’t much obvious value to the work, maybe the work—not the measurement of the work—needs to change. (19:49) Smart leaders know that deriving a simple calculation of indirect contributions is complex—otherwise, the topic wouldn’t keep coming up. There is a “why” behind why they’re asking, and when you understand the “why,” you’ll be better positioned to deliver the value they actually seek, using valuation measurements that are “just enough” in their precision. What do you think it says to a stakeholder if you’re spending an inordinate amount of time simply trying to calculate and explain the value of your data product? (23:22) Many organizations for years have invested in things that don’t always have a short term ROI. They know that ROI takes time, and they can’t really measure what it’s worth along the way. Examples include investments in company culture, innovation, brand reputation, and many others. If you’re constantly playing defense and having to justify your existence or methods by quantifying the financial value of your data products (or data product management team, or UX team, or any other indirect contributor/contribution), then either your work truly does lack value, or you haven’t surfaced what the actual success metrics and outcomes are— in the eyes of the stakeholder. As such, the perceived value is “low” or opaque. They might be looking for a hard number to assign to it because they’re not seeing any of the other forms of value that they care about that would indicate positive progress. It’s easier to write [you] a large check for a big, innovative, unproven initiative if your stakeholders know what you and your team can accomplish with a small check. (35:16) Links Experiencing Data: Episode 80 with Doug Hubbard…
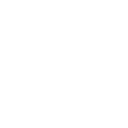
1 162 - Beyond UI: Designing User Experiences for LLM and GenAI-Based Products 42:07
42:07
Play Later
Play Later
Lists
Like
Liked42:07
I’m doing things a bit differently for this episode of Experiencing Data. For the first time on the show, I’m hosting a panel discussion. I’m joined by Thomson Reuters’s Simon Landry, Sumo Logic’s Greg Nudelman, and Google’s Paz Perez to chat about how we design user experiences that improve people’s lives and create business impact when we expose LLM capabilities to our users. With the rise of AI, there are a lot of opportunities for innovation, but there are also many challenges—and frankly, my feeling is that a lot of these capabilities right now are making things worse for users, not better. We’re looking at a range of topics such as the pros and cons of AI-first thinking, collaboration between UX designers and ML engineers, and the necessity of diversifying design teams when integrating AI and LLMs into b2b products. Highlights/ Skip to Thoughts on how the current state of LLMs implementations and its impact on user experience (1:51) The problems that can come with the "AI-first" design philosophy (7:58) Should a company's design resources be spent on go toward AI development? (17:20) How designers can navigate "fuzzy experiences” (21:28) Why you need to narrow and clearly define the problems you’re trying to solve when building LLMs products (27:35) Why diversity matters in your design and research teams when building LLMs (31:56) Where you can find more from Paz, Greg, and Simon (40:43) Quotes from Today’s Episode “ [AI] will connect the dots. It will argue pro, it will argue against, it will create evidence supporting and refuting, so it’s really up to us to kind of drive this. If we understand the capabilities, then it is an almost limitless field of possibility. And these things are taught, and it’s a fundamentally different approach to how we build user interfaces. They’re no longer completely deterministic. They’re also extremely personalized to the point where it’s ridiculous.” - Greg Nudelman (12:47) “ To put an LLM into a product means that there’s a non-zero chance your user is going to have a [negative] experience and no longer be your customer. That is a giant reputational risk, and there’s also a financial cost associated with running these models. I think we need to take more of a service design lens when it comes to [designing our products with AI] and ask what is the thing somebody wants to do… not on my website, but in their lives? What brings them to my [product]? How can I imagine a different world that leverages these capabilities to help them do their job? Because what [designers] are competing against is [a customer workflow] that probably worked well enough.” - Simon Landry (15:41) “ When we go general availability (GA) with a product, that traditionally means [designers] have done all the research, got everything perfect, and it’s all great, right? Today, GA is a starting gun. We don’t know [if the product is working] unless we [seek out user feedback]. A massive research method is needed. [We need qualitative research] like sitting down with the customer and watching them use the product to really understand what is happening[…] but you also need to collect data. What are they typing in? What are they getting back? Is somebody who’s typing in this type of question always having a short interaction? Let’s dig into it with rapid, iterative testing and evaluation, so that we can update our model and then move forward. Launching a product these days means the starting guns have been fired. Put the research to work to figure out the next step.” - (23:29) Greg Nudelman “ I think that having diversity on your design team (i.e. gender, level of experience, etc.) is critical. We’ve already seen some terrible outcomes. Multiple examples where an LLM is crafting horrendous emails, introductions, and so on. This is exactly why UXers need to get involved [with building LLMs]. This is why diversity in UX and on your tech team that deals with AI is so valuable. Number one piece of advice: get some researchers. Number two: make sure your team is diverse.” - Greg Nudelman (32:39) “ It’s extremely important to have UX talks with researchers, content designers, and data teams. It’s important to understand what a user is trying to do, the context [of their decisions], and the intention. [Designers] need to help [the data team] understand the types of data and prompts being used to train models. Those things are better when they’re written and thought of by [designers] who understand where the user is coming from. [Design teams working with data teams] are getting much better results than the [teams] that are working in a vacuum.” - Paz Perez (35:19) Links Milly Barker’s LinkedIn post Greg Nudelman’s Value Matrix Article Greg Nudelman website Paz Perez on Medium Paz Perez on LinkedIn Simon Landry LinkedIn…
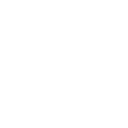
1 161 - Designing and Selling Enterprise AI Products [Worth Paying For] 34:00
34:00
Play Later
Play Later
Lists
Like
Liked34:00
With GenAI and LLMs comes great potential to delight and damage customer relationships—both during the sale, and in the UI/UX. However, are B2B AI product teams actually producing real outcomes, on the business side and the UX side, such that customers find these products easy to buy, trustworthy and indispensable? What is changing with customer problems as a result of LLM and GenAI technologies becoming more readily available to implement into B2B software? Anything? Is your current product or feature development being driven by the fact you might be able to now solve it with AI? The “AI-first” team sounds like it’s cutting edge, but is that really determining what a customer will actually buy from you? Today I want to talk to you about the interplay of GenAI, customer trust (both user and buyer trust), and the role of UX in products using probabilistic technology. These thoughts are based on my own perceptions as a “user” of AI “solutions,” (quotes intentional!), conversations with prospects and clients at my company (Designing for Analytics), as well as the bright minds I mentor over at the MIT Sandbox innovation fund. I also wrote an article about this subject if you’d rather read an abridged version of my thoughts. Highlights/ Skip to: AI and LLM-Powered Products Do Not Turn Customer Problems into “Now” and “Expensive” Problems (4:03) Trust and Transparency in the Sale and the Product UX: Handling LLM Hallucinations (Confabulations) and Designing for Model Interpretability (9:44) Selling AI Products to Customers Who Aren’t Users (13:28) How LLM Hallucinations and Model Interpretability Impact User Trust of Your Product (16:10) Probabilistic UIs and LLMs Don’t Negate the Need to Design for Outcomes (22:48) How AI Changes (or Doesn’t) Our Benchmark Use Cases and UX Outcomes (28:41) Closing Thoughts (32:36) Quotes from Today’s Episode “Putting AI or GenAI into a product does not change the urgency or the depth of a particular customer problem; it just changes the solution space. Technology shifts in the last ten years have enabled founders to come up with all sorts of novel ways to leverage traditional machine learning, symbolic AI, and LLMs to create new products and disrupt established products; however, it would be foolish to ignore these developments as a product leader. All this technology does is change the possible solutions you can create. It does not change your customer situation, problem, or pain, either in the depth, or severity, or frequency. In fact, it might actually cause some new problems. I feel like most teams spend a lot more time living in the solution space than they do in the problem space. Fall in love with the problem and love that problem regardless of how the solution space may continue to change.” (4:51) “Narrowly targeted, specialized AI products are going to beat solutions trying to solve problems for multiple buyers and customers. If you’re building a narrow, specific product for a narrow, specific audience, one of the things you have on your side is a solution focused on a specific domain used by people who have specific domain experience. You may not need a trillion-parameter LLM to provide significant value to your customer. AI products that have a more specific focus and address a very narrow ICP I believe are more likely to succeed than those trying to serve too many use cases—especially when GenAI is being leveraged to deliver the value. I think this can be true even for platform products as well. Narrowing the audience you want to serve also narrows the scope of the product, which in turn should increase the value that you bring to that audience—in part because you probably will have fewer trust, usability, and utility problems resulting from trying to leverage a model for a wide range of use cases.” (17:18) “Probabilistic UIs and LLMs are going to create big problems for product teams, particularly if they lack a set of guiding benchmark use cases. I talk a lot about benchmark use cases as a core design principle and data-rich enterprise products. Why? Because a lot of B2B and enterprise products fall into the game of ‘adding more stuff over time.’ ‘Add it so you can sell it.’ As products and software companies begin to mature, you start having product owners and PMs attached to specific technologies or parts of a product. Figuring out how to improve the customer’s experience over time against the most critical problems and needs they have is a harder game to play than simply adding more stuff— especially if you have no benchmark use cases to hold you accountable. It’s hard to make the product indispensable if it’s trying to do 100 things for 100 people.“ (22:48) “Product is a hard game, and design and UX is by far not the only aspect of product that we need to get right. A lot of designers don’t understand this, and they think if they just nail design and UX, then everything else solves itself. The reason the design and experience part is hard is that it’s tied to behavior change– especially if you are ‘disrupting’ an industry, incumbent tool, application, or product. You are in the behavior-change game, and it’s really hard to get it right. But when you get it right, it can be really amazing and transformative.” (28:01) “If your AI product is trying to do a wide variety of things for a wide variety of personas, it’s going to be harder to determine appropriate benchmarks and UX outcomes to measure and design against. Given LLM hallucinations, the increased problem of trust, model drift problems, etc., your AI product has to actually innovate in a way that is both meaningful and observable to the customer. It doesn’t matter what your AI is trying to “fix.” If they can’t see what the benefit is to them personally, it doesn’t really matter if technically you’ve done something in a new and novel way. They’re just not going to care because that question of what’s in it for me is always sitting behind, in their brain, whether it’s stated out loud or not.” (29:32) Links Designing for Analytics mailing list…
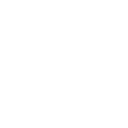
1 160 - Leading Product Through a Merger/Acquisition: Lessons from The Predictive Index’s CPO Adam Berke 42:10
42:10
Play Later
Play Later
Lists
Like
Liked42:10
Today, I’m chatting with Adam Berke, the Chief Product Officer at The Predictive Index. For 70 years, The Predictive Index has helped customers hire the right employees, and after the merger with Charma, their products now nurture the employee/manager relationship. This is something right up Adam’s alley, as he previously helped co-found the employee and workflow performance management software company Charma before both aforementioned organizations merged back in 2023. You’ll hear Adam talk about the first-time challenges (and successes) that come with integrating two products and two product teams, and why squashing out any ambiguity with overindexing (i.e. coming prepared with new org charts ASAP) is essential during the process. Integrating behavioral science into the world of data is what has allowed The Predictive Index to thrive since the 1950s. While this is the company’s main selling point, Adam explains how the science-forward approach can still create some disagreements–and learning opportunities–with The Predictive Index’s legacy customers. Highlights/ Skip to: What is The Predictive Index and how does the product team conduct their work (1:24) Why Charma merged with The Predictive Index (5:11) The challenges Adam has faced as a CPO since the Charma/Predictive Index merger (9:21) How Predictive Index has utilized behavioral science to remove the guesswork of hiring (14:22) The makeup of the product team that designs and delivers The Predictive Index's products (20:24) Navigating the clashes between changing science and Predictive Index's legacy customers (22:37) How The Predictive Index analyzes the quality of their products with multiple user data metrics (27:21) What Adam would do differently if had to redo the merger (37:52) Where you can find more from Adam and The Predictive Index (41:22) Quotes from Today’s Episode “ Acquisitions are complicated. Outside of a few select companies, there are very few that have mergers and acquisitions as a repeatable discipline. More often than not, neither [company in the merger] has an established playbook for how to do this. You’re [acquiring a company] because of its product, team, or maybe even one feature. You have different theories on how the integration might look, but experiencing it firsthand is a whole different thing. My initial role didn’t exist in [The Predictive Index] before. The rest of the whole PI organization knows how to get their work done before this, and now there’s this new executive. There’s just tons of [questions and confusion] if you don’t go in assuming good faith and be willing to work through the bumps. It’s going to get messy.” - Adam Berke (9:41) “We integrated the teams and relaunched the product. Charma became [a part of the product called] PI Perform, and right away there was re-skinning, redesign, and some back-end architecture that needed to happen to make it its own module. From a product perspective, we’re trying to deliver [Charma’s] unique value prop. That’s when we can start [figuring out how to] infuse PI’s behavioral science into these workflows. We have this foundation. We got the thing organized. We got the teams organized. We were 12 people when we were acquired… and here we are a year later. 150+ new customers have been added to PI Perform because it’s accelerating now that we’re figuring out the product.” - Adam Berke (12:18) “Our product team has the roles that you would expect: a PM, researcher, ux design, and then one atypical role–a PhD behavioral scientist. [Our product already had] suggested topics and templates [for manager/IC one-on-one meetings], but now we want to make those templates and suggested topics more dynamic. There might be different questions to draw out a better discussion, and our behavioral scientists help us determine [those questions]... [Our behavioral scientists] look at the science, other research, and calibrate [the one-on-one questions] before we implement them into the product.” - Adam Berke (21:04) “We’ve adapted the technology and science over time as they move forward. We want to update the product with the most recent science, but there are customers who have used this product in a certain way for decades in some cases. Our desire is to follow the science… but you can’t necessarily stop people from using the stuff in a way that they used it 20 years ago. We sometimes end up with disagreements [with customers over product changes based on scientific findings], and those are tricky conversations. But even in that debate… it comes down to all the best practices you would follow in product development in general–listening to your customers, asking that additional ‘why’ question, and trying to get to root causes.” - Adam Berke (23:36) “ We’re doing an upgrade to our platform right now trying to figure out how to manage user permissions in the new version of the product. The way that we did it in the old version had a lot of problems associated… and we put out a survey. “Hey, do you use this to do X?’ We got hundreds of responses and found that half of them were not using it for the reason that we thought they were. At first, we thought thousands of people were going to have deep, deep sensitivities to tweaks in how this works, and now we realize that it might be half that, at best. A simple one-question survey asked about the right problem in the right way can help to avoid a lot of unnecessary thrashing on a product problem that might not have even existed in the first place.” - Adam Berke (35:22) Links Referenced The Predictive Index: https://www.predictiveindex.com/ LinkedIn: https://www.linkedin.com/in/adamberke/…
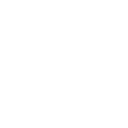
1 159 - Uncorking Customer Insights: How Data Products Revealed Hidden Gems in Liquor & Hospitality Retail 40:47
40:47
Play Later
Play Later
Lists
Like
Liked40:47
Today, I’m talking to Andy Sutton, GM of Data and AI at Endeavour Group, Australia's largest liquor and hospitality company. In this episode, Andy—who is also a member of the Data Product Leadership Community (DPLC)—shares his journey from traditional, functional analytics to a product-led approach that drives their mission to leverage data and personalization to build the “Spotify for wines.” This shift has greatly transformed how Endeavour’s digital and data teams work together, and Andy explains how their advanced analytics work has paid off in terms of the company’s value and profitability. You’ll learn about the often overlooked importance of relationships in a data-driven world, and how Andy sees the importance of understanding how users do their job in the wild (with and without your product(s) in hand). Earlier this year, Andy also gave the DPLC community a deeper look at how they brew data products at EDG, and that recording is available to our members in the archive. We covered: What it was like at EDG before Andy started adopting a producty approach to data products and how things have now changed (1:52) The moment that caused Andy to change how his team was building analytics solutions (3:42) The amount of financial value that Andy's increased with his scaling team as a result of their data product work (5:19) How Andy and Endeavour use personalization to help build “the Spotify of wine” (9:15) What the team under Andy required in order to make the transition to being product-led (10:27) The successes seen by Endeavour through the digital and data teams’ working relationship (14:04) What data product management looks like for Andy’s team (18:45) How Andy and his team find solutions to bridging the adoption gap (20:53) The importance of exposure time to end users for the adoption of a data product (23:43) How talking to the pub staff at EDG’s bars and restaurants helps his team build better data products (27:04) What Andy loves about working for Endeavour Group (32:25) What Andy would change if he could rewind back to 2022 and do it all over (34:55) Final thoughts (38:25) Quotes from Today’s Episode “I think the biggest thing is the value we unlock in terms of incremental dollars, right? I’ve not worked in analytics team before where we’ve been able to deliver a measurable value…. So, we’re actually—in theory—we’re becoming a profit center for the organization, not just a cost center. And so, there’s kind of one key metric. The second one, we do measure the voice of the team and how engaged our team are, and that’s on an upward trend since we moved to the new operating model, too. We also measure [a type of] “voice of partner” score [and] get something like a 4.1 out of 5 on that scale. Those are probably the three biggest ones: we’re putting value in, and we’re delivering products, I guess, our internal team wants to use, and we are building an enthused team at the same time.” - Andy Sutton (16:18) “ You can put an [unfinished] product in front of an end customer, and they will give you quality feedback that you can then iterate on quickly. You can do that with an internal team, but you’ll lose credibility. Internal teams hold their analytics colleagues to a higher standard than the external customers. We’re trying to change how people do their roles. People feel very passionate about the roles they do, and how they do them, and what they bring to that role. We’re trying to build some of that into products. It requires probably more design consideration than I’d anticipated, and we’re still bringing in more designers to help us move closer to the start line.’” - Andy Sutton (19:25) “ [Customer research] is becoming critical in terms of the products we’re building. You’re building a product, a set of products, or a process for an operations team. In our context, an operations team can mean a team of people who run a pub. It’s not just about convincing me, my product managers, or my data scientists that you need research; we want to take some of the resources out of running that bar for a period of time because we want to spend time with [the pub staff] watching, understanding, and researching. We’ve learned some of these things along the way… we’ve earned the trust, we’ve earned that seat at the table, and so we can have those conversations. It’s not trivial to get people to say, ‘I’ll give you a day-long workshop, or give you my team off of running a restaurant and a bar for the day so that they can spend time with you, and so you can understand our processes.’” - Andy Sutton (24:42) “ I think what is very particular to pubs is the importance of the interaction between the customer and the person serving the customer. [Pubs] are about the connections between the staff and the customer, and you don’t get any of that if you’re just looking at things from a pure data perspective… You don’t see the [relationships between pub staff and customer] in the [data], so how do you capture some of that in your product? It’s about understanding the context of the data, not just the data itself.” - Andy Sutton (28:15) “Every winery, every wine grower, every wine has got a story. These conversations [and relationships] are almost natural in our business. Our CEO started work on the shop floor in one of our stores 30 years ago. That kind of relationship stuff percolates through the organization. Having these conversations around the customer and internal stakeholders in the context of data feels a lot easier because storytelling and relationships are the way we get things done. An analytics team may get frustrated with people who can’t understand data, but it’s [the analytics team’s job] to help bridge that gap.” - Andy Sutton (32:34) Links Referenced LinkedIn: https://www.linkedin.com/in/andysutton/ Endeavour Group: https://www.endeavourgroup.com.au/ Data Product Leadership Community https://designingforanalytics.com/community…
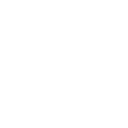
1 158 - From Resistance to Reliance: Designing Data Products for Non-Believers with Anna Jacobson of Operator Collective 43:41
43:41
Play Later
Play Later
Lists
Like
Liked43:41
After getting started in construction management, Anna Jacobson traded in the hard hat for the world of data products and operations at a VC company. Anna, who has a structural engineering undergrad and a masters in data science, is also a Founding Member of the Data Product Leadership Community (DPLC). However, her work with data products is more “accidental” and is just part of her responsibility at Operator Collective. Nonetheless, Anna had a lot to share about building data products, dashboards, and insights for users—including resistant ones! That resistance is precisely what I wanted to talk to her about in this episode: how does Anna get somebody to adopt a data product to which they may be apathetic, if not completely resistant? At the end of the episode, Anna gives us a sneak peek at what she’s planning to talk about in our final 2024 live DPLC group discussion coming up on 12/18/2024. We covered: (1:17) Anna's background and how she got involved with data products (3:32) The ways Anna applied her experiences working in construction management to her current work with data products at a VC firm (5:32) Explaining one of the main data products she works on at Operator Collective (9:55) How Anna defines success for her data products (15:21) The process of designing data products for "non-believers" (21:08) How to think about "super users" and their feedback on a data product (27:11) How a company's cultural problems can be a blocker for product adoption (38:21) A preview of what you can expect from Anna's talk and live group discussion in the DPLC (40:24) Closing thoughts from Anna (42:54) Where you can find more from Anna Quotes from Today’s Episode “People working with data products are always thinking about how to [gain user adoption of their product]... I can’t think of a single one where [all users] were immediately on board. There’s a lot to unpack in what it takes to get non-believers on board, and it’s something that none of us ever get any training on. You just learn through experience, and it’s not something that most people took a class on in college. All of the social science around what we do gets really passed over for all the technical stuff. It takes thinking through and understanding where different [users] are coming from, and [understanding] that my perspective alone is not enough to make it happen.” - Anna Jacobson (16:00) “If you only bring together the super users and don’t try to get feedback from the average user, you are missing the perspective of the person who isn’t passionate about the product. A non-believer is someone who is just over capacity. They may be very hard-working, they may be very smart, but they just don’t have the bandwidth for new things. That’s something that has to be overcome when you’re putting a new product into place.” - Anna Jacobson (22:35) “If a company can’t find budget to support [a data product], that’s a cultural decision. It’s not a financial decision. They find the money for the things that they care about. Solving the technology challenge is pretty easy, but you have to have a company that’s motivated to do that. If you want to implement something new, be it a data product or any change in an organization, identifying the cultural barriers and figuring out how to bring [people in an organization] on board is the crux of it. The money and the technology can be found.” - Anna Jacobson (27:58) “I think people are actually very bad at explaining what they want, and asking people what they want is not helpful. If you ask people what they want to do, then I think you have a shot at being able to build a product that does [what they want]. The executive sponsors typically have a very different perspective on what the product [should be] than the users do. If all of your information is getting filtered through the executive sponsor, you’re probably not getting the full picture” - Anna Jacobson (31:45) “You want to define what the opportunity is, the problem, the solution, and you want to talk about costs and benefits. You want to align [the data product] with corporate strategy, and those things are fairly easy to map out. But as you get down to the user, what they want to know is, ‘How is this going to make my life easier? How is this going to make [my job] faster? How is it going to result in better outcomes?’ They may have an interest in how it aligns with corporate strategy, but that’s not what’s going to motivate them. It’s really just easier, faster, better.” - Anna Jacobson (35:00) Links Referenced LinkedIn: https://www.linkedin.com/in/anna-ching-jacobson/ DPLC (Data Product Leadership Community): https://designingforanalytics.com/community…
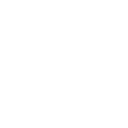
1 157 - How this materials science SAAS company brings PM+UX+data science together to help materials scientists accelerate R&D 34:58
34:58
Play Later
Play Later
Lists
Like
Liked34:58
R&D for materials-based products can be expensive, because improving a product’s materials takes a lot of experimentation that historically has been slow to execute. In traditional labs, you might change one variable, re-run your experiment, and see if the data shows improvements in your desired attributes (e.g. strength, shininess, texture/feel, power retention, temperature, stability, etc.). However, today, there is a way to leverage machine learning and AI to reduce the number of experiments a material scientist needs to run to gain the improvements they seek. Materials scientists spend a lot of time in the lab—away from a computer screen—so how do you design a desirable informatics SAAS that actually works, and fits into the workflow of these end users? As the Chief Product Officer at MaterialsZone, Ori Yudilevich came on Experiencing Data with me to talk about this challenge and how his PM, UX, and data science teams work together to produce a SAAS product that makes the benefits of materials informatics so valuable that materials scientists depend on their solution to be time and cost-efficient with their R&D efforts. We covered: (0:45) Explaining what Ori does at MaterialZone and who their product serves (2:28) How Ori and his team help make material science testing more efficient through their SAAS product (9:37) How they design a UX that can work across various scientific domains (14:08) How “doing product” at MaterialsZone matured over the past five years (17:01) Explaining the "Wizard of Oz" product development technique (21:09) The importance of integrating UX designers into the "Wizard of Oz" (23:52) The challenges MaterialZone faces when trying to get users to adopt to their product (32:42) Advice Ori would've given himself five years ago (33:53) Where you can find more from MaterialsZone and Ori Quotes from Today’s Episode “The fascinating thing about materials science is that you have this variety of domains, but all of these things follow the same process. One of the problems [consumer goods companies] face is that they have to do lengthy testing of their products. This is something you can use machine learning to shorten. [Product research] is an iterative process that typically takes a long time. Using your data effectively and using machine learning to predict what can happen, what’s better to try out, and what will reduce costs can accelerate time to market.” - Ori Yudilevich (3:47) “The difference [in time spent testing a product] can be up to 70% [i.e. you can run 70% fewer experiments using ML.] That [also] means 70% less resources you’re using. Under the ‘old system’ of trial and error, you were just trying out a lot of things. The human mind cannot process a large number of parameters at once, so [a materials scientist] would just start playing only with [one parameter at a time]. You’ll have many experiments where you just try to optimize [for] one parameter, but then you might have 20, 30, or 100 more [to test]. Using machine learning, you can change a lot of parameters at once. The model can learn what has the most effect, what has a positive effect, and what has a negative effect. The differences can be really huge.” - Ori Yudilevich (5:50) “Once you go deeper into a use case, you see that there are a lot of differences. The types of raw materials, the data structure, the quantity of data, etc. For example, with batteries, you have lots of data because you can test hundreds all at once. Whereas with something like ceramics, you don’t try so many [experiments]. You just can’t. It’s much slower. You can’t do so many [experiments] in parallel. You have much less data. Your models are different, and your data structure is different. But there’s also quite a lot of commonality because you’re storing the data. In the end, you have each domain, some raw materials, formulations, tests that you’re doing, and different statistical plots that are very common.” - Ori Yudilvech (11:24) “We’ll typically do what we call the ‘Wizard of Oz’ technique. You simulate as if you have a feature, but you’re actually working for your client behind the scenes. You tell them [the simulated feature] is what you’re doing, but then measure [the client’s response] to understand if there’s any point in further developing that feature. Once you validate it, have enough data, and know where the feature is going, then you’ll start designing it and releasing it in incremental stages. We’ve made a lot of progress in how we discover opportunities and how we build something iteratively to make sure that we’re always going in the right direction” - Ori Yudilevich (15:56) “The main problem we’re encountering is changing the mindset of users. Our users are not people who sit in front of a computer. These are researchers who work in [a materials science] lab. The challenge [we have] is getting people to use the platform more. To see it’s worth [their time] to look at some insights, and run the machine learning models. We’re always looking for ways to make that transition faster… and I think the key is making [the user experience] just fun, easy, and intuitive.” - Ori Yudilevich (24:17) “Even if you make [the user experience] extremely smooth, if [users] don’t see what they get out of it, they’re still not going to [adopt your product] just for the sake of doing it. What we find is if this [product] can actually make them work faster or develop better products– that gets them interested. If you’re adopting these advanced tools, it makes you a better researcher and worker. People who [adopt those tools] grow faster. They become leaders in their team, and they slowly drag the others in.” - Ori Yudilevich (26:55) “Some of [MaterialsZone’s] most valuable employees are the people who have been users. Our product manager is a materials scientist. I’m not a material scientist, and it’s hard to imagine being that person in the lab. What I think is correct turns out to be completely wrong because I just don’t know what it’s like. Having [material scientists] who’ve made the transition to software and data science? You can’t replace that.” - Ori Yudilevich (31:32) Links Referenced Website: https://www.materials.zone LinkedIn: https://www.linkedin.com/in/oriyudilevich/ Email: ori@materials.zone…
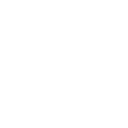
1 156-The Challenges of Bringing UX Design and Data Science Together to Make Successful Pharma Data Products with Jeremy Forman 41:37
41:37
Play Later
Play Later
Lists
Like
Liked41:37
Jeremy Forman joins us to open up about the hurdles– and successes that come with building data products for pharmaceutical companies. Although he’s new to Pfizer, Jeremy has years of experience leading data teams at organizations like Seagen and the Bill and Melinda Gates Foundation. He currently serves in a more specialized role in Pfizer’s R&D department, building AI and analytical data products for scientists and researchers. . Jeremy gave us a good luck at his team makeup, and in particular, how his data product analysts and UX designers work with pharmaceutical scientists and domain experts to build data-driven solutions.. We talked a good deal about how and when UX design plays a role in Pfizer’s data products, including a GenAI-based application they recently launched internally. Highlights/ Skip to: (1:26) Jeremy's background in analytics and transition into working for Pfizer (2:42) Building an effective AI analytics and data team for pharma R&D (5:20) How Pfizer finds data products managers (8:03) Jeremy's philosophy behind building data products and how he adapts it to Pfizer (12:32) The moment Jeremy heard a Pfizer end-user use product management research language and why it mattered (13:55) How Jeremy's technical team members work with UX designers (18:00) The challenges that come with producing data products in the medical field (23:02) How to justify spending the budget on UX design for data products (24:59) The results we've seen having UX design work on AI / GenAI products (25:53) What Jeremy learned at the Bill & Melinda Gates Foundation with regards to UX and its impact on him now (28:22) Managing the "rough dance" between data science and UX (33:22) Breaking down Jeremy's GenAI application demo from CDIOQ (36:02) What would Jeremy prioritize right now if his team got additional funding (38:48) Advice Jeremy would have given himself 10 years ago (40:46) Where you can find more from Jeremy Quotes from Today’s Episode “We have stream-aligned squads focused on specific areas such as regulatory, safety and quality, or oncology research. That’s so we can create functional career pathing and limit context switching and fragmentation. They can become experts in their particular area and build a culture within that small team. It’s difficult to build good [pharma] data products. You need to understand the domain you’re supporting. You can’t take somebody with a financial background and put them in an Omics situation. It just doesn’t work. And we have a lot of the scars, and the failures to prove that.” - Jeremy Forman (4:12) “You have to have the product mindset to deliver the value and the promise of AI data analytics. I think small, independent, autonomous, empowered squads with a product leader is the only way that you can iterate fast enough with [pharma data products].” - Jeremy Forman (8:46) “The biggest challenge is when we say data products. It means a lot of different things to a lot of different people, and it’s difficult to articulate what a data product is. Is it a view in a database? Is it a table? Is it a query? We’re all talking about it in different terms, and nobody’s actually delivering data products.” - Jeremy Forman (10:53) “I think when we’re talking about [data products] there’s some type of data asset that has value to an end-user, versus a report or an algorithm. I think it’s even hard for UX people to really understand how to think about an actual data product. I think it’s hard for people to conceptualize, how do we do design around that? It’s one of the areas I think I’ve seen the biggest challenges, and I think some of the areas we’ve learned the most. If you build a data product, it’s not accurate, and people are getting results that are incomplete… people will abandon it quickly.” - Jeremy Forman (15:56) “ I think that UX design and AI development or data science work is a magical partnership, but they often don’t know how to work with each other. That’s been a challenge, but I think investing in that has been critical to us. Even though we’ve had struggles… I think we’ve also done a good job of understanding the [user] experience and impact that we want to have. The prototype we shared [at CDIOQ] is driven by user experience and trying to get information in the hands of the research organization to understand some portfolio types of decisions that have been made in the past. And it’s been really successful.” - Jeremy Forman (24:59) “If you’re having technology conversations with your business users, and you’re focused only the technology output, you’re just building reports. [After adopting If we’re having technology conversations with our business users and only focused on the technology output, we’re just building reports. [After we adopted a human-centered design approach], it was talking [with end-users] about outcomes, value, and adoption. Having that resource transformed the conversation, and I felt like our quality went up. I felt like our output went down, but our impact went up. [End-users] loved the tools, and that wasn’t what was happening before… I credit a lot of that to the human-centered design team.” - Jeremy Forman (26:39) “When you’re thinking about automation through machine learning or building algorithms for [clinical trial analysis], it becomes a harder dance between data scientists and human-centered design. I think there’s a lack of appreciation and understanding of what UX can do. Human-centered design is an empathy-driven understanding of users’ experience, their work, their workflow, and the challenges they have. I don’t think there’s an appreciation of that skill set.” - Jeremy Forman (29:20) “Are people excited about it? Is there value? Are we hearing positive things? Do they want us to continue? That’s really how I’ve been judging success. Is it saving people time, and do they want to continue to use it? They want to continue to invest in it. They want to take their time as end-users, to help with testing, helping to refine it. Those are the indicators. We’re not generating revenue, so what does the adoption look like? Are people excited about it? Are they telling friends? Do they want more? When I hear that the ten people [who were initial users] are happy and that they think it should be rolled out to the whole broader audience, I think that’s a good sign.” - Jeremy Forman (35:19) Links Referenced LinkedIn: https://www.linkedin.com/in/jeremy-forman-6b982710/…
Welcome to Player FM!
Player FM is scanning the web for high-quality podcasts for you to enjoy right now. It's the best podcast app and works on Android, iPhone, and the web. Signup to sync subscriptions across devices.