Player FM - Internet Radio Done Right
Checked 24d ago
הוסף לפני three שנים
תוכן מסופק על ידי Klaviyo Data Science Team. כל תוכן הפודקאסטים כולל פרקים, גרפיקה ותיאורי פודקאסטים מועלים ומסופקים ישירות על ידי Klaviyo Data Science Team או שותף פלטפורמת הפודקאסט שלהם. אם אתה מאמין שמישהו משתמש ביצירה שלך המוגנת בזכויות יוצרים ללא רשותך, אתה יכול לעקוב אחר התהליך המתואר כאן https://he.player.fm/legal.
Player FM - אפליקציית פודקאסט
התחל במצב לא מקוון עם האפליקציה Player FM !
התחל במצב לא מקוון עם האפליקציה Player FM !
Klaviyo Data Science EP 19 | 2021: A Data Science Year in Review
Manage episode 317314457 series 3251385
תוכן מסופק על ידי Klaviyo Data Science Team. כל תוכן הפודקאסטים כולל פרקים, גרפיקה ותיאורי פודקאסטים מועלים ומסופקים ישירות על ידי Klaviyo Data Science Team או שותף פלטפורמת הפודקאסט שלהם. אם אתה מאמין שמישהו משתמש ביצירה שלך המוגנת בזכויות יוצרים ללא רשותך, אתה יכול לעקוב אחר התהליך המתואר כאן https://he.player.fm/legal.
…
continue reading
58 פרקים
Manage episode 317314457 series 3251385
תוכן מסופק על ידי Klaviyo Data Science Team. כל תוכן הפודקאסטים כולל פרקים, גרפיקה ותיאורי פודקאסטים מועלים ומסופקים ישירות על ידי Klaviyo Data Science Team או שותף פלטפורמת הפודקאסט שלהם. אם אתה מאמין שמישהו משתמש ביצירה שלך המוגנת בזכויות יוצרים ללא רשותך, אתה יכול לעקוב אחר התהליך המתואר כאן https://he.player.fm/legal.
…
continue reading
58 פרקים
כל הפרקים
×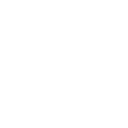
1 Klaviyo Data Science Podcast EP 58 | All Aboard the Leadership 48:22
48:22
הפעל מאוחר יותר
הפעל מאוחר יותר
רשימות
לייק
אהבתי48:22
All successful teams have at least one leader, and most have at least one manager. This episode, we dive into how leadership works on highly technical teams, how managing a highly technical team works, and why the two aren’t exactly the same thing. Listen along for more discussion about: The traits of highly effective leaders — and how that might look different on an engineering or data science team How to know that a move into management fits you Our guests’ best recommendations for management books, resources, and experiences For more details, including links to the many resources our panel suggest to learn more about leadership and management, check out the full writeup on Medium !…
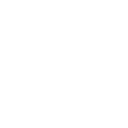
1 Klaviyo Data Science Podcast EP 57 | Agile, or, Don't Go Chasing Waterfall 44:53
44:53
הפעל מאוחר יותר
הפעל מאוחר יותר
רשימות
לייק
אהבתי44:53
What is agile methodology — and, just as importantly, what is it not? Whether you’re new to agile entirely or you stay up late pondering its most philosophical inner workings, if you want to know more about agile and how organizations can reap its benefits while avoiding its pitfalls, this is the episode for you. You’ll learn about a variety of topics, including: How to effectively compromise between the tenets of agile and the realities of building software — and how not to When agile helps you pivot, and what that means for your customers Our guests’ hottest takes about agile For the full show notes, including who's who, see the Medium writeup .…
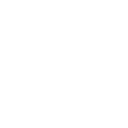
1 Klaviyo Data Science Podcast EP 56 | Evaluating AI Models: A Seminar (feat. Evan Miller) 45:29
45:29
הפעל מאוחר יותר
הפעל מאוחר יותר
רשימות
לייק
אהבתי45:29
This month, the Klaviyo Data Science Podcast welcomes Evan Miller to deliver a seminar on his recently published paper, Adding Error Bars to Evals: A Statistical Approach to Language Model Evaluations ! This episode is a mix of a live seminar Evan gave to the team at Klaviyo and an interview we conducted with him afterward. Suppose you’re trying to understand the performance of an AI model — maybe one you built or fine-tuned and are comparing to state-of-the-art models, maybe one you’re considering loading up and using for a project you’re about to start. If you look at the literature today, you can get a sense of what the average performance for the model is on an evaluation or set of tasks. But often, that’s unfortunately the extent of what it’s possible to learn —there is much less emphasis placed on the variability or uncertainty inherent to those estimates. And as anyone who’s worked with a statistical model in the past can affirm, variability is a huge part of why you might choose to use or discard a model. This seminar explores how to best compute, summarize, and display estimates of variability for AI models. Listen along to hear about topics like: Why the Central Limit Theorem you learned about in Stats 101 is still relevant with the most advanced AI models developed today How to think about complications of classic assumptions, such as measurement error or clustering, in the AI landscape When to do a sample size calculation for your AI model, and how to do it About Evan Miller You may already know our guest Evan Miller from his fantastic blog , which includes his celebrated A/B testing posts, such as “How not to run an A/B test.” You may also have used his A/B testing tools , such as the sample size calculator. Evan currently works as a research scientist at Anthropic. About Anthropic Per Anthropic’s website : You can find more information about Anthropic, including links to their social media accounts, on the company website. Anthropic is an AI safety and research company based in San Francisco. Our interdisciplinary team has experience across ML, physics, policy, and product. Together, we generate research and create reliable, beneficial AI systems. Special thanks to Chris Murphy at Klaviyo for organizing this seminar and making this episode possible! For the full show notes, including who's who, see the Medium writeup .…
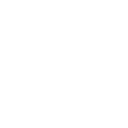
1 Klaviyo Data Science Podcast EP 55 | 2024 Year in Review 49:55
49:55
הפעל מאוחר יותר
הפעל מאוחר יותר
רשימות
לייק
אהבתי49:55
Welcome back to the Klaviyo Data Science podcast! This episode, we dive into… 2024 Year in Review As the new year starts, we take a look back at 2024. We spoke to data scientists and people who work closely with data scientists, and we asked them all the question we ask every year: what is the coolest data science thing you learned about in 2024? You’ll hear a wide range of answers, including: How a rhyme can topple LLM security Why the Sequential Probably Ratio Test is better at measuring basketball ability than the NBA playoffs How badly a non-specialized LLM could beat you at chess For the full show notes, including who's who, see the Medium writeup .…
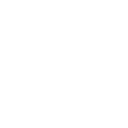
1 Klaviyo Data Science Podcast EP 54| The Right to Exclude 41:13
41:13
הפעל מאוחר יותר
הפעל מאוחר יותר
רשימות
לייק
אהבתי41:13
How we protect invention and ingenuity: Patents Writing software often involves taking known patterns, combining and shaping them, and adding needed context or specialization related to the problem we’re trying to solve. Sometimes, that means writing something that’s effectively been written by someone else before. But sometimes, that means creating something new. What should you do in a case where you’ve genuinely created something new? Perhaps more importantly, how do you know when you’re in that situation? This month, we explore one of the best tools to help answer both questions: the patent process. Listen along with your fearless co-hosts and a member of Klaviyo’s legal team to learn about what a patent is, why getting them matters, and how to get your own novel work patented, along with: Tips to keep in mind when applying for a patent Knowing which software you should try to patent How to get your organization to buy into patents For the full show notes, including who's who, see the Medium writeup .…
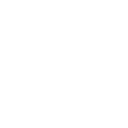
1 Klaviyo Data Science Podcast EP 53 | Yahoogle 48:21
48:21
הפעל מאוחר יותר
הפעל מאוחר יותר
רשימות
לייק
אהבתי48:21
Welcome to the November episode of the Klaviyo Data Science Podcast for 2024! In years past, November episodes reflected the chaotic Black Friday/Cyber Monday season by examining unique challenges of readiness, scale, and fundamental changes happening with little to no warning, as well as how those challenges were handled; this November is no different. What happens when two of the largest email platforms make sweeping changes to their spam filters, providing a few short months of notice? Stress, uncertainty, and an opportunity for individuals and organization to rise to the challenge. In this month’s episode, we talk with analysts, engineers, and product managers to discuss Klaviyo’s journey to meet Yahoo and Google’s new Email Delivery Requirements — aka Yahoogle, the colloqial name for a new set of rules that must be followed by email senders to have their emails make it to inboxes and not go straight to the junk bin. Listen in to hear more about: Yahoogle. It’s more than just a funny word! The challenges in operating a large-scale email sending system Advice and retrospectives about large, cross-functional projects and tight deadlines For the full show notes, including who's who, see the Medium writeup .…
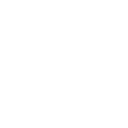
1 Klaviyo Data Science Podcast EP 52 | Our Favorite Models and How They Work 51:08
51:08
הפעל מאוחר יותר
הפעל מאוחר יותר
רשימות
לייק
אהבתי51:08
As most data scientists will tell you, there is no such thing as the single best model or the perfect model . Some work well in some circumstances but poorly in others, some present a specific tradeoff between factors like flexibility and explainability that is only useful in certain settings. Some are best set up to handle specific types of data that don’t arise in every single project. But at the same time, most data scientists would acknowledge that some models manage to stand out. Maybe it’s nostalgia, maybe it’s how powerful they are in some settings, maybe it’s another factor entirely — but for one reason or another, most data scientists will admit they have a soft spot for some models. That’s what we’re here to discuss this month: what is your favorite model? Listen in to hear more about: The benefits of simple models How learning about some models teaches you about entire fields of mathematics What fitting a classification model has to teach us about epistemology For the full show notes, including who's who, see the Medium writeup .…
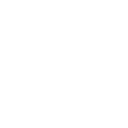
1 Klaviyo Data Science Podcast EP 51 | How Personalization Empowers Users 44:10
44:10
הפעל מאוחר יותר
הפעל מאוחר יותר
רשימות
לייק
אהבתי44:10
If you’re making software, especially data science-powered software, there’s a good chance one of your biggest goals is to empower stronger and deeper personalization for your users. Our topic for this month: how can you do even more than that? How can we make personalization not just robust, but both more effective and easier than the alternative? It’s not a simple task, but it is one that the team we interviewed this month has tackled. Listen in to hear more about: Why personalization is a matter of finishing your user’s… sandwiches How to approach complex personalization features as a data scientist, a designer, or an engineer What the game of Battleship can teach you about personalization For the full show notes, including who's who, see the Medium writeup .…
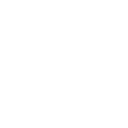
1 Klaviyo Data Science Podcast EP 50 | The 50th Episode Celebration Special 44:40
44:40
הפעל מאוחר יותר
הפעל מאוחר יותר
רשימות
לייק
אהבתי44:40
It may come as a suprise to those of you reading this, but this milestone snuck up on me. I was surprised to realize we’d reached a full 50 episodes. What better time to take a moment to reflect and look back? This episode is all about the Klaviyo Data Science Podcast. We talk through the history of the podcast, how we approach making episodes that matter to our listeners, our highlight episodes, and what we’ve learned through the years. You’ll hear about: Why you don’t need a fancy mic to get started How to approach talking about deep, technical work on the air What we have planned for the next 50 episodes For the full show notes, including who's who, see the Medium writeup .…
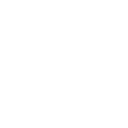
1 Klaviyo Data Science Podcast EP 49 | What Real Data Scientists Wish They'd Known Earlier in their Careers 43:30
43:30
הפעל מאוחר יותר
הפעל מאוחר יותר
רשימות
לייק
אהבתי43:30
A big part of growing and developing as a data scientist, or any other member of a data science team, is taking time to reflect, learn, and distill experiences into advice. This month, we’ve asked four senior members of the data science team to do exactly that: look back over their careers, reflect on what they know and what they wished they’d known earlier, and tell everyone what those lessons are. Listen to this advice-filled episode to hear: How taking a more scenic or indirect career route can impart valuable experiences The growth and development opportunities that truly unlocked new phases of their careers, and how you can make the most of similar opportunities Why mistakes often make the best learning opportunities For the full show notes, including who's who, see the Medium writeup .…
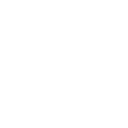
1 Klaviyo Data Science Podcast EP 48 | Data Science Goes Worldwide 42:36
42:36
הפעל מאוחר יותר
הפעל מאוחר יותר
רשימות
לייק
אהבתי42:36
Internationalizing your product There are many aspects of product growth — reaching new heights for peak volume, reaching new levels of sustained daily volume, growing your feature set and the complexity of your code based, and many others. Dealing with growth in an intelligent and forward-looking way is never easy, but this month we deal with a type of growth that presents its own unique set of challenges: international growth, i.e. expanding the range of countries and languages your products are natively available in. This month, we talked with multiple members of the internationalization effort here at Klaviyo, from teams across our organization. You’ll hear about: How strings can be far more complex than they seem Why changing languages doesn’t just mean changing words Where in the world your assumptions about language may break For the full show notes, including who's who, see the Medium writeup .…
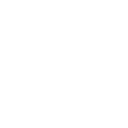
1 Klaviyo Data Science Podcast EP 47 | Cooking Up Something Special with Data Science: Made In Cookware 48:10
48:10
הפעל מאוחר יותר
הפעל מאוחר יותר
רשימות
לייק
אהבתי48:10
How real marketers use data science We spend a lot of time on this podcast talking about how to build data science solutions. Implicit in many of those conversations is perhaps the most fundamental truth of product design and development: we build data science solutions because people use them. We aren’t doing this just for fun — the reason we spend so much time, effort, and energy to refine our solutions is that it actually matters to real people. This month, we talk to some of those people. In particular, we sat down with two members of the team at Made In Cookware ( http://madeincookware.com/ ) to discuss what makes their business unique, how they approach understanding and marketing to their customers, and how data science and AI help them do all of that. You’ll hear about: What kitchen knives can teach you about product design and development Which type of pan you should use to cook a steak, and how that can help you understand customer segmentation How AI saves real marketers real time while also giving them better results About Made In Made In Cookware (Made In) is a premium cookware brand based in Austin, TX. Founded in 2017 but born of a 4th-generation, family-owned kitchen supply business, Made In creates best-in-class cookware developed in partnership with the world’s finest chefs and foremost craftsmen. Today, you’ll find Made In products in more than 2,000 restaurants, in the hands of James Beard Award-winning chefs at Michelin-starred restaurants across the country, and in the kitchens of home cooks everywhere. Made In products have garnered over 100,000 5-star reviews, and the company was named one of Inc. Magazine’s best workplaces and Newsweek’s best online shops of 2024. For the full show notes, including who's who, see the Medium writeup .…
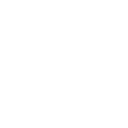
1 Klaviyo Data Science Podcast EP 46 | ML Ops 101 45:10
45:10
הפעל מאוחר יותר
הפעל מאוחר יותר
רשימות
לייק
אהבתי45:10
An Introduction to ML Ops Building data science products requires many things we’ve discussed on this podcast before: insight, customer empathy, strategic thinking, flexibility, and a whole lot of determination. But it requires one more thing we haven’t talked about nearly as much: a stable, performant, and easy-to-use foundation. Setting up that foundation is the chief goal of the field of machine learning operations, aka ML Ops. This month on the Klaviyo Data Science Podcast, we give a brief but thorough introduction to the field of ML Ops. You’ll hear about: How ML Ops is different from the similar fields of data science and DevOps What skills a successful ML Ops developer should have, and what an ML Ops developer’s day-to-day looks like Why concepts like “velocity” and “stability” have their own special nuances in the world of ML Ops For the full show notes, including who's who, see the Medium writeup .…
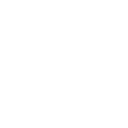
1 Klaviyo Data Science Podcast EP 45 | SegmentsAI: An AI Case Study on Delivering Value 42:14
42:14
הפעל מאוחר יותר
הפעל מאוחר יותר
רשימות
לייק
אהבתי42:14
In many ways, 2023 was the year of AI in tech, which is a double-edged sword. On the one hand, the basic technology is straightforwardly exciting — but on the other hand, with seemingly every technology solution scrambling to integrate a thin wrapper around ChatGPT, it’s hard to stand out in a saturated environment. This month on the Klaviyo Data Science Podcast, we dive into a case study of how to build AI products, SegmentsAI, and discuss the principles that go into making sure your AI-powered product shines — and, more importantly, actually helps your customers. You’ll hear about: How to know when AI is the right solution for the problem The unique technical challenges that come with building an AI product, from user testing to validation The answer to the AI chicken-and-egg problem “Why do this, why build another LLM feature? It seems like every website is rushing to get their name next to AI... How you break through the noise is to actually provide value to people, not novelty. Being able to help customers speed up or generate new, interesting segments that they otherwise wouldn’t? I think that’s valuable.”— Rob Huselid, Senior Data Scientist For the full show notes, including who's who, see the Medium writeup .…
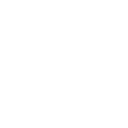
1 Klaviyo Data Science Podcast EP 44 | The Data Powering EDI 52:17
52:17
הפעל מאוחר יותר
הפעל מאוחר יותר
רשימות
לייק
אהבתי52:17
Welcome back to the Klaviyo Data Science podcast! This episode, we dive into… Equity, Diversity, and Inclusion Equity, diversity, and inclusion (EDI) are more than just central principles of successful teams in data science and beyond — they’re also a rich field that presents interesting and challenging data science problems. This episode, we chat with two EDI specialists at Klaviyo about EDI, the data that powers it, and the challenges that come with using that data. You’ll hear about: Why EDI is a core part of both processes and products How to work with self-reported data — and, sometimes, work around the fact that you don’t actually have the data you want Examples of EDI work in action For the full show notes, including who's who, see the Medium writeup .…
ברוכים הבאים אל Player FM!
Player FM סורק את האינטרנט עבור פודקאסטים באיכות גבוהה בשבילכם כדי שתהנו מהם כרגע. זה יישום הפודקאסט הטוב ביותר והוא עובד על אנדרואיד, iPhone ואינטרנט. הירשמו לסנכרון מנויים במכשירים שונים.