An award-winning cannabis podcast for women, by women. Hear joyful stories and useful advice about cannabis for health, well-being, and fun—especially for needs specific to women like stress, sleep, and sex. We cover everything from: What’s the best weed for sex? Can I use CBD for menstrual cramps? What are the effects of the Harlequin strain or Gelato strain? And, why do we prefer to call it “cannabis” instead of “marijuana”? We also hear from you: your first time buying legal weed, and how ...
…
continue reading
Player FM - Internet Radio Done Right
11 subscribers
Checked 3d ago
הוסף לפני six שנים
תוכן מסופק על ידי UCTV. כל תוכן הפודקאסטים כולל פרקים, גרפיקה ותיאורי פודקאסטים מועלים ומסופקים ישירות על ידי UCTV או שותף פלטפורמת הפודקאסט שלהם. אם אתה מאמין שמישהו משתמש ביצירה שלך המוגנת בזכויות יוצרים ללא רשותך, אתה יכול לעקוב אחר התהליך המתואר כאן https://he.player.fm/legal.
Player FM - אפליקציית פודקאסט
התחל במצב לא מקוון עם האפליקציה Player FM !
התחל במצב לא מקוון עם האפליקציה Player FM !
פודקאסטים ששווה להאזין
בחסות
<
<div class="span index">1</div> <span><a class="" data-remote="true" data-type="html" href="/series/series-2996722">The So What from BCG</a></span>


This podcast from Boston Consulting Group looks around the corner of today’s big business and social issues. The goal–the so what–is to make sense of today and prepare busy leaders and executives for the day after tomorrow. Award-winning British journalist Georgie Frost interviews the leading thinkers and doers at BCG on the trends, developments, and ideas that will shape and disrupt the future. This is not your typical business strategy podcast. This podcast uses the following third-party services for analysis: Podtrac - https://analytics.podtrac.com/privacy-policy-gdrp
How to Create AI to Solve Real-World Problems
Manage episode 367016239 series 2578515
תוכן מסופק על ידי UCTV. כל תוכן הפודקאסטים כולל פרקים, גרפיקה ותיאורי פודקאסטים מועלים ומסופקים ישירות על ידי UCTV או שותף פלטפורמת הפודקאסט שלהם. אם אתה מאמין שמישהו משתמש ביצירה שלך המוגנת בזכויות יוצרים ללא רשותך, אתה יכול לעקוב אחר התהליך המתואר כאן https://he.player.fm/legal.
This series on artificial intelligence explores recent breakthroughs of AI, its broader societal implications and its future potential. In this presentation, Sergey Levine, associate professor of Electrical Engineering and Computer Science at UC Berkeley, discusses AI reinforcement learning methods. Levine asks what it would take to create machine learning systems that can make decisions when faced with the full complexity and diversity of the real world, while still retaining the ability of reinforcement learning to come up with new solutions? He discusses how advances in offline reinforcement learning can enable machine learning systems to learn to make more optimal decisions from data, combining the best of data-driven machine learning with the capacity for emergent behavior and optimization provided by reinforcement learning. Levine received a BS and MS in Computer Science from Stanford University in 2009, and a Ph.D. in Computer Science from Stanford University in 2014. He joined the faculty of the Department of Electrical Engineering and Computer Sciences at UC Berkeley in fall 2016. His work focuses on machine learning for decision making and control, with an emphasis on deep learning and reinforcement learning algorithms. Applications of his work include autonomous robots and vehicles, as well as applications in other decision-making domains. His research includes developing algorithms for end-to-end training of deep neural network policies that combine perception and control, scalable algorithms for inverse reinforcement learning, deep reinforcement learning algorithms, and more. Series: "Data Science Channel" [Science] [Show ID: 38857]
…
continue reading
81 פרקים
Manage episode 367016239 series 2578515
תוכן מסופק על ידי UCTV. כל תוכן הפודקאסטים כולל פרקים, גרפיקה ותיאורי פודקאסטים מועלים ומסופקים ישירות על ידי UCTV או שותף פלטפורמת הפודקאסט שלהם. אם אתה מאמין שמישהו משתמש ביצירה שלך המוגנת בזכויות יוצרים ללא רשותך, אתה יכול לעקוב אחר התהליך המתואר כאן https://he.player.fm/legal.
This series on artificial intelligence explores recent breakthroughs of AI, its broader societal implications and its future potential. In this presentation, Sergey Levine, associate professor of Electrical Engineering and Computer Science at UC Berkeley, discusses AI reinforcement learning methods. Levine asks what it would take to create machine learning systems that can make decisions when faced with the full complexity and diversity of the real world, while still retaining the ability of reinforcement learning to come up with new solutions? He discusses how advances in offline reinforcement learning can enable machine learning systems to learn to make more optimal decisions from data, combining the best of data-driven machine learning with the capacity for emergent behavior and optimization provided by reinforcement learning. Levine received a BS and MS in Computer Science from Stanford University in 2009, and a Ph.D. in Computer Science from Stanford University in 2014. He joined the faculty of the Department of Electrical Engineering and Computer Sciences at UC Berkeley in fall 2016. His work focuses on machine learning for decision making and control, with an emphasis on deep learning and reinforcement learning algorithms. Applications of his work include autonomous robots and vehicles, as well as applications in other decision-making domains. His research includes developing algorithms for end-to-end training of deep neural network policies that combine perception and control, scalable algorithms for inverse reinforcement learning, deep reinforcement learning algorithms, and more. Series: "Data Science Channel" [Science] [Show ID: 38857]
…
continue reading
81 פרקים
כל הפרקים
×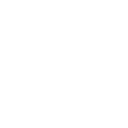
1 The Moral Economy of Resource Extraction and the Future of Industrialization 1:20:24
1:20:24
הפעל מאוחר יותר
הפעל מאוחר יותר
רשימות
לייק
אהבתי1:20:24
The "energy transition" is actually a shift from relying on fossil fuels (like coal, oil, and gas) to using metals to generate energy. However, extracting metals has always been a significant environmental and political issue, especially for cities. This problem has been around for centuries, even ancient Roman writers wrote about it. In this program, Helen Thompson, Professor of Political Economy at Cambridge University, talks about the historic use of fossil fuels and its economic, social and environmental impacts to the transition today to extracting metals for energy, dominated by China. Thompson points out that extracting resources will always have environmental and social costs. To mitigate these risks, she says we need to find ways to reduce international competition and ecological damage. This requires acknowledging that the idea of endless progress, which was fueled by fossil fuels, has its limits. And she says we must prioritize sustainability and responsible resource management to create a better future. Series: "UC Berkeley Graduate Lectures" [Public Affairs] [Business] [Show ID: 40428]…
A look at the iconic Sather Gate at UC Berkeley. Series: "UC Landmarks" [Arts and Music] [Show ID: 40500]
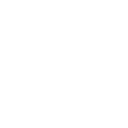
1 Forging a New Political System 2024 and Beyond 1:29:40
1:29:40
הפעל מאוחר יותר
הפעל מאוחר יותר
רשימות
לייק
אהבתי1:29:40
Historian and political commentator Heather Cox Richardson joins UC Berkeley professor of law and history Dylan Penningroth in a timely conversation about the reshaping of the United States’ two major political parties. A professor of 19th century American history at Boston College, Richardson provides an incisive perspective on current politics to the more than three million readers of her nightly newsletter, Letters from an American. She has written for the Washington Post, the New York Times, and the Guardian, and is the author, most recently, of the best-selling book "Democracy Awakening: Notes on the State of America." Penningroth is the author of the award-winning "Before the Movement: The Hidden History of Black Civil Rights." Series: "UC Berkeley Graduate Lectures" [Public Affairs] [Show ID: 40424]…
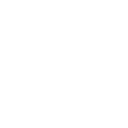
1 The Arc of Energy Justice: A Pursuit to Ensure Affordable Reliable and Clean Energy for All 52:13
52:13
הפעל מאוחר יותר
הפעל מאוחר יותר
רשימות
לייק
אהבתי52:13
We are at a critical moment in our society. While we advance efforts to mitigate and adapt to the climate crisis, across the globe, millions are experiencing issues of energy affordability, reliability and equitable access to modern energy technologies. In this program, Tony Reames, Professor of Environmental Justice at the University of Michigan School for Environment and Sustainability, explores the intricate intersection of energy, class, race and place, shedding light on inequities in access to and the distribution of energy resources. Reames discusses how various factors, including socio-economic conditions, policy landscapes and environmental characteristics contribute to energy inequities. The talk underscores the importance of understanding and addressing these issues in the pursuit of energy justice, emphasizing the need for inclusive scholarship, policies and funding that empower marginalized communities. Series: "UC Berkeley Graduate Lectures" [Public Affairs] [Science] [Show ID: 40223]…
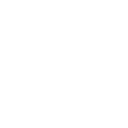
1 Do Cash Transfers Save Lives? 1:11:51
1:11:51
הפעל מאוחר יותר
הפעל מאוחר יותר
רשימות
לייק
אהבתי1:11:51
Does giving cash up front improve the health and wellbeing of people in poor communities? In this program, Edward (Ted) Miguel, professor of economics and co-director of the Center for Effective Global Action at UC Berkeley, talks about his work in Kenya on the impact of cash transfers on infant mortality, leveraging a unique large-scale census of local households’ birth histories. The findings provide novel evidence on the broader impacts of cash transfers on wellbeing of a poor rural population, and illustrate the value of the experimental approach in development economics for public policy. Series: "UC Berkeley Graduate Lectures" [Public Affairs] [Show ID: 40309]…
This program explores the decolonizing potential of Indian aesthetic-social philosophy by challenging two entrenched colonial prejudices: the supposed radical dissimilarity and inferiority of pre-modern Indian traditions compared to modern social theory. Through an analysis of the Upanishads and Vaisnava theology and poetry, Sudipta Kaviraj, professor of Indian Politics and Intellectual History at Columbia University, examines conceptions of paradise as a life without suffering, arguing that divergent ideas of paradise have shaped Indian aesthetic thought. Central to this philosophy is the interdependence of cognitive curiosity and aesthetic enjoyment, seen as essential for fully accessing and understanding the universe. Kaviraj suggests that these traditions offer valuable insights for modern secular thinkers reflecting on the human condition. Series: "UC Berkeley Graduate Lectures" [Humanities] [Show ID: 40222]…
What defines a person’s character, and how does it shape who they are? In this lecture, Susan Wolf, emeritus professor of philosophy at the University of North Carolina, challenges traditional ideas about character. She argues that character is more than just a set of traits or values an individual endorses—it can include aspects of ourselves we may not even recognize or approve of. Wolf explores how a deeper understanding of character, rooted in active intelligence and thoughtful reflection, can reshape how we view agency, moving beyond just actions and intentions. This thought-provoking talk offers fresh insights into what makes us who we are and how we navigate the complexities of identity and selfhood. Series: "UC Berkeley Graduate Lectures" [Humanities] [Show ID: 40221]…
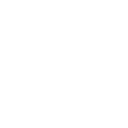
1 The Deadly Trade in Oil and Gas 1:27:32
1:27:32
הפעל מאוחר יותר
הפעל מאוחר יותר
רשימות
לייק
אהבתי1:27:32
Oil and gas are the most traded commodities on the planet; they are also the chief causes of the most grievous harm our species has yet faced, the burgeoning climate crisis. Bill McKibben is the Schumann Distinguished Scholar at Middlebury College and a founder of Third Act, which organizes people over the age of 60 to work on climate and racial justice. He examines how the export of hydrocarbons, in particular, has become an enormous threat to efforts to rein in greenhouse gasses. It explores the role that America – the world’s biggest exporter of gas – plays in this ongoing catastrophe. And it looks at the role that non-tradeable commodities – sunshine and wind – play in easing this crisis. Series: "UC Berkeley Graduate Lectures" [Science] [Show ID: 40220]…
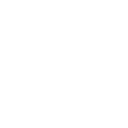
1 The Future of American Democracy: The 2024 Election and Beyond 1:13:45
1:13:45
הפעל מאוחר יותר
הפעל מאוחר יותר
רשימות
לייק
אהבתי1:13:45
As voters prepare to head to the polls on Election Day, join the Goldman School of Public Policy and Cal Performances for a critical look at the moment we’re in, the issues that have shaped and led us to this year’s tumultuous election, and the future of American democracy. UC Berkeley experts from former presidential administrations—Janet Napolitano, former Secretary of Homeland Security under the Obama administration (2009-2013); Robert Reich, former Secretary of Labor under the Clinton administration (1993-1997); and Maria Echaveste, former Assistant to the President and Deputy White House Chief of Staff under the Clinton Administration (1998-2001)—as well as PolicyLink founder-in-residence and Chief Vision Officer for the Goldman School of Public Policy’s new Democracy Policy Initiative, Angela Glover Blackwell. Series: "The Goldman School - Berkeley Public Policy" [Public Affairs] [Show ID: 40302]…
This program aims to recover Plato’s idea of craft or art, Greek technê, in the expansive sense which includes not only the handicrafts but skilled practices from housebuilding to navigation. Rachel Barney, professor of philosophy at the University of Toronto, examines Plato and other Greek thinkers who were fascinated by the craft model: the idea that both the moral virtue of the good person and the political widom of the expert ruler are — or could be made into — skilled practices as reliable as shoemaking or carpentry. Similar ideas appear in classical Chinese philosophy, developed in very different ways by Daoist and Confucian thinkers. In our time, craft is in a bad way: marginalized in theory and everywhere endangered in practice. Ancient thinkers can help us to see what remains valuable and urgent about craft today, and what a reinvigorated understanding of it might contribute to our ethical and political thought. Crafts to be considered include carpentry, medicine, drawing, film editing, the ‘multicraft’ of the restaurant, tennis, and traditional Polynesian navigation. Philosophical points of reference, in addition to Plato, Aristotle, Zhuangzi, and Xunzi, include Murdoch, MacIntyre, Korsgaard, and the Hart-Fuller debate, as well as literary reflections from Kazuo Ishiguro and Cormac McCarthy. Barney is joined by Adam Gopnik, Rachana Kamtekar, Christine Korsgaard, and Alexander Nehamas to discuss the topic of craft. Series: "Tanner Lectures on Human Values" [Humanities] [Business] [Show ID: 39865]…
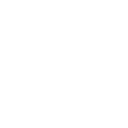
1 Housing and Homelessness in California 38:45
38:45
הפעל מאוחר יותר
הפעל מאוחר יותר
רשימות
לייק
אהבתי38:45
Across the United States, homelessness has been on the rise. In California, there have been over 181,000 people without a stable place to call home—about 30 percent of the nation’s homeless population. During the COVID-19 pandemic, those numbers continued to rise as earnings dropped and the housing affordability crisis worsened. What interventions have prevented people from becoming homeless? What lessons have we learned from local, regional, and statewide efforts to reduce unsheltered homelessness in the Bay Area and beyond? The Terner Center for Housing Innovation, the Goldman School of Public Policy, and a diverse panel of cross-sector experts and advocates collaborated for a discussion on reducing poverty and addressing homelessness in California. Series: "The Goldman School - Berkeley Public Policy" [Public Affairs] [Show ID: 39849]…
What is a craft? For Plato, paradigmatic craft-practitioners include the doctor, carpenter and navigator; an updated, more generous conception should include the dancer, coder, waitress, painter, chef, professional athlete, and firefighter. Rachel Barney, professor of philosophy at the University of Toronto, discusses how each of these skilled practices is oriented to the achievement of a distinctive end, the goodness of which is independent of the self-interest or inclinations of the practitioner. This Platonic conception of craft as involving disinterested teleological rationality can explain how craft sets objective norms for correct action, and for the excellence of the practitioner. And it shows that to master a craft is not merely to acquire knowledge or skills but to take on the ‘internal standpoint’ definitive of the craft, internalizing its values and treating its reasons for action as authoritative. Barney is joined by Adam Gopnik and Rachana Kamtekar for commentary on the topic of craft. Series: "Tanner Lectures on Human Values" [Humanities] [Business] [Show ID: 39863]…
Especially when practiced as a line of work — as a job or métier — craft sets norms for its practitioners. On the whole, a shoemaker should try to be a good shoemaker, and the good person who is a shoemaker routinely does just that. But what kind of ‘should’ is this, and what could connect these two kinds of goodness? Rachel Barney, professor of philosophy at the University of Toronto, examines prominent philosophical conceptions of craft, ancient and modern, offer wildly various explanations of its normative authority. The picture is complicated by the way in which craft-as-work is paradigmatic both for successful practical reason and for social roles or practical identities in general. But the most fundamental source of craft’s normativity is the one which Plato and Aristotle bring out: the fact that, when practised as a job or métier, practicing your craft can be a way to realize the human good. And so thinking about craft turns out to be a way of thinking about Utopia: a society in which a just distribution of work could secure both the flourishing of the worker and the common good. Barney is joined by Christine Korsgaard and Alexander Nehamas for commentary on the topic of craft. Series: "Tanner Lectures on Human Values" [Humanities] [Business] [Show ID: 39864]…
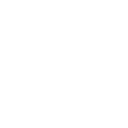
1 American Thanatocracy vs Abolition Democracy: On Cops Capitalism and the War on Black Life 1:34:43
1:34:43
הפעל מאוחר יותר
הפעל מאוחר יותר
רשימות
לייק
אהבתי1:34:43
In this program, Robin D. G. Kelley, Distinguished Professor and Gary B. Nash Endowed Chair in U.S. History at UCLA, examines how police in the neoliberal era–in tandem with other state and corporate entities—have become engines of capital accumulation, government revenue, gentrification, the municipal bond market, the tech and private security industry—in a phrase, the profits of death. Kelley argues the police don’t just take lives; they make life and living less viable for the communities they occupy. The growth of police power has also fundamentally weakened democracy and strengthened “thanatocracy”—rule by death– especially with respect to Black communities. Kelley says these same communities have produced a new abolition democracy, organizing to advance a different future, without oppression and exploitation, war, poverty, prisons, police, borders, the constraints of imposed gender, sexual, and ableist norms, and an economic system that destroys the planet while generating obscene inequality. Series: "UC Berkeley Graduate Lectures" [Public Affairs] [Humanities] [Show ID: 39780]…
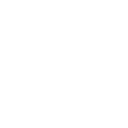
1 How the Supreme Court Divided America 57:13
57:13
הפעל מאוחר יותר
הפעל מאוחר יותר
רשימות
לייק
אהבתי57:13
The 2021-2022 term of the U.S. Supreme Court is widely considered to be the most consequential in living memory. Bruen, West Virginia v. EPA, Dobbs—the Court’s rulings in these controversial cases weakened gun restrictions, hobbled the Environmental Protection Agency’s ability to fight climate change, and overturned the constitutional protection for abortion rights nearly 50 years after Roe v. Wade. In The Supermajority: How the Supreme Court Divided America, Brennan Center for Justice president Michael Waldman examines the term’s major cases, the meaning of “originalism”—a new, extreme method of interpreting the Constitution—and offers proposals for reform. Join Waldman and Maria Echaveste, President and CEO of the Opportunity Institute and former senior White House official, for an in-depth look at the tumultuous 2021-2022 term and a discussion of how these decisions will affect every American for generations to come. Series: "The Goldman School - Berkeley Public Policy" [Public Affairs] [Show ID: 39848]…
U
UC Berkeley (Video)

1 American Democracy and the Crisis of Majority Rule 59:49
59:49
הפעל מאוחר יותר
הפעל מאוחר יותר
רשימות
לייק
אהבתי59:49
America’s contemporary democratic predicament is rooted in its historically incomplete democratization. Born in a pre-democratic era, the constitution’s balancing of majority rule and minority rights created still-unresolved dilemmas. Placing the U.S. in comparative perspective, Daniel Ziblatt, professor of government at Harvard University, offers new perspectives on what should be “beyond the reach of majorities” – and what should not – making the case for a fuller democracy as antidote to the perils of our age. Ziblatt is also director of the Transformations of Democracy group at Berlin’s WZB Social Science Center. He is the author of four books, including "How Democracies Die," co-authored with Steve Levitsky, a New York Times best-seller. His newest book co-authored with Steven Levitsky is entitled "Tyranny of the Minority." Series: "UC Berkeley Graduate Lectures" [Public Affairs] [Show ID: 39237]…
U
UC Berkeley (Video)

1 'I' and Self-Consciousness 1:53:29
1:53:29
הפעל מאוחר יותר
הפעל מאוחר יותר
רשימות
לייק
אהבתי1:53:29
What does it mean when we use the first-person pronoun ‘I’? And how does it relate to self-consciousness? In this program, Béatrice Longuenesse, professor of philosophy emerita at New York University, compares the analysis of philosophers Elizabeth Anscombe and Jean-Paul Sartre on consciousness, self-consciousness and the use of 'I'. Languenesse's current work spans the history of philosophy, especially Kant and nineteenth century German philosophy; the philosophy of language and mind; and philosophical issues related to Freudian psychanalysis. Series: "UC Berkeley Graduate Lectures" [Humanities] [Show ID: 39240]…
U
UC Berkeley (Video)

1 Policies to Restore the American Dream with Raj Chetty 1:21:30
1:21:30
הפעל מאוחר יותר
הפעל מאוחר יותר
רשימות
לייק
אהבתי1:21:30
Where did the American Dream of hard work equals upward mobility go? And what will it take to bring it back? In this talk, Raj Chetty, director of Opportunity Insights and professor of public economics at Harvard University, focuses on three policy levers to increase upward mobility: reducing racial and economic segregation through more effective affordable housing programs, investing in place-based policies, and strengthening higher education. Chetty gives specific examples of pilot studies and interventions that help inform the design of policy and practice from the federal to state to local levels, including at institutions of higher education such as UC Berkeley. He offers illustrations that can be scaled nationally, providing a pathway to expand opportunities for all. Series: "UC Berkeley Graduate Lectures" [Public Affairs] [Business] [Show ID: 39239]…
U
UC Berkeley (Video)

1 The Science of Economic Opportunity: New Insights from Big Data with Raj Chetty 1:27:38
1:27:38
הפעל מאוחר יותר
הפעל מאוחר יותר
רשימות
לייק
אהבתי1:27:38
Children’s chances of earning more than their parents have fallen from 90% to 50% over the past half century in America. How can we restore the American Dream of upward mobility for all children? In this talk, Raj Chetty, director of Opportunity Insights and professor of public economics at Harvard University, shows how big data from varied sources ranging from anonymized tax records to Facebook social network data is helping us uncover the science of economic opportunity. Among other topics, Chetty discusses how and why children’s chances of climbing the income ladder vary across neighborhoods, the drivers of racial disparities in economic mobility, and the role of social capital as a driver of upward mobility. He presents data on the state of economic opportunity in California in particular to provide a local context to these national patterns. Series: "UC Berkeley Graduate Lectures" [Public Affairs] [Business] [Show ID: 39238]…
U
UC Berkeley (Video)

1 Imitation and Innovation in AI: What 4-Year-Olds Can Do and AI Can’t (Yet) 58:18
58:18
הפעל מאוחר יותר
הפעל מאוחר יותר
רשימות
לייק
אהבתי58:18
Young children’s learning may be an important model for artificial intelligence (AI). In this program, Alison Gopnik, professor of psychology and member of the Berkeley Artificial Intelligence Research (BAIR) Lab at UC Berkeley, says that comparing children and artificial agents in the same tasks and environments can help us understand the abilities of existing systems and create new ones. In particular, many current large data-supervised systems, such as large language models (LLMs), provide new ways to access information collected by past agents. However, they lack the kinds of exploration and innovation that are characteristic of children. New techniques may help to instantiate childlike curiosity, exploration and play in AI systems. This program is co-hosted with the UC Berkeley College of Computing, Data Science, and Society and the UC Berkeley Artificial Intelligence Research (BAIR) Lab. About the Series: CITRIS Research Exchange delivers fresh perspectives on information technology and society from distinguished academic, industry and civic leaders. Free and open to the public, these seminars feature leading voices on societal-scale research issues. Series: "Data Science Channel" [Science] [Show ID: 39351]…
U
UC Berkeley (Video)

Researchers used to define objectives for artificial intelligence (AI) agents by hand, but with progress in optimization and reinforcement learning, it became obvious that it's too difficult to think of everything ahead of time and write it down. Instead, these days the objective is viewed as a hidden part of the state on which researchers can receive feedback or observations from humans — how they act and react, how they compare options, what they say. In this talk, Anca Dragan, Associate Professor of Electrical Engineering and Computer Sciences at UC Berkeley, discusses what this transition has achieved, what open challenges researchers still face and ideas for mitigating them. Dragan discusses applications in robotics and how the lessons there apply to virtual agents like large language models. Series: "Data Science Channel" [Science] [Show ID: 39350]…
U
UC Berkeley (Video)

1 Underappreciated Evergreen Companies: Capitalism at Its Best with David Whorton 1:47:50
1:47:50
הפעל מאוחר יותר
הפעל מאוחר יותר
רשימות
לייק
אהבתי1:47:50
After founding four companies and working at top firms in venture capital and private equity, where fast growth and maximum profits rule, David Whorton, Founder and CEO of the Tugboat Institute, has spent the last decade exploring and developing the concept of the evergreen company—one built to last privately over 100 years. The evergreen company stands in contrast to those that are being built to flip to generate wealth for a small few. Instead, evergreen companies are being built with very long planning horizons and the commitment to share their success with their employees and their communities. Whorton argues evergreen companies are incredibly important to our society, but overlooked and under-appreciated relative to venture capitalists, private equity and public companies that represent the de facto growth company models. Since the dot.com boom, the de facto growth model for venture capitalists has been get-big-fast. It later evolved to growth-at-all-costs with the advent of cheap money under loose Fed policies. This play book led to numerous excesses, including the manic pursuit of ever larger and higher valuation rounds in hot companies. In the same period, private equity has risen dramatically, unwisely seen by many as a safer asset class than public stocks; an industry sits on over a trillion dollars of dry powder to invest, matched with a couple trillion of debt, giving the private equity firms purchasing power over $3 trillion dollars. Series: "Tanner Lectures on Human Values" [Business] [Show ID: 39235]…
U
UC Berkeley (Video)

1 A Conversation with Ezra Klein about Liberalism 1:26:49
1:26:49
הפעל מאוחר יותר
הפעל מאוחר יותר
רשימות
לייק
אהבתי1:26:49
California’s deepest problems — the skyrocketing cost of housing, the lagging development of clean energy, the traffic choking the state — reflect an inability of Democratic governments to build real things in the real world quickly and affordably. The result is liberal governance that routinely fails to achieve liberal outcomes. New York Times opinion columnist and podcast host Ezra Klein talks with Amy E. Lerman, Chair and Professor of Public Policy and Political Science at UC Berkeley, about how we got here and what can be done about it. Series: "UC Berkeley Graduate Lectures" [Public Affairs] [Business] [Show ID: 39236]…
U
UC Berkeley (Video)

1 Data Dignity and the Inversion of AI 47:22
47:22
הפעל מאוחר יותר
הפעל מאוחר יותר
רשימות
לייק
אהבתי47:22
In this program, Jaron Lanier, Microsoft's prime unifying scientist, discusses a piece he published in The New Yorker (“There Is No AI”) about applying data dignity ideas to artificial intelligence. Lanier argues that large-model AI can be reconceived as a social collaboration by the people who provide data to the model in the form of text, images and other modalities. This is a figure/ground inversion of the usual conception of AI as being a participant or collaborator in its own right. Explanations of model results and behaviors would then center around the relative influence of specific inputs through a provenance calculation mechanism. This formulation suggests new and different strategies for long-term economics in the context of high-performance AI, as well as more concrete approaches to many safety, fairness and alignment questions. This program is co-hosted with the UC Berkeley College of Computing, Data Science, and Society and the UC Berkeley Artificial Intelligence Research (BAIR) Lab. The CITRIS Research Exchange delivers fresh perspectives on information technology and society from distinguished academic, industry and civic leaders. Series: "Data Science Channel" [Science] [Show ID: 39326]…
U
UC Berkeley (Video)

1 Debunking Trust and Safety: Unveiling the Reality Behind Online Integrity with Yoel Roth 29:02
29:02
הפעל מאוחר יותר
הפעל מאוחר יותר
רשימות
לייק
אהבתי29:02
This episode of TecHype features Yoel Roth, former Head of Trust and Safety at Twitter. Yoel provides first-hand insights into how one of the largest online platforms in the world built out its trust and safety operations to better ensure its service was helpful, harmless, and aligned with user expectations While at Twitter, Dr. Roth found himself the target of a coordinated harassment campaign on the platform, one instigated by the current CEO Elon Musk. His years of work building out the trust and safety operations had become personal. In this episode, Dr. Roth provides professional and personal perspectives on the real benefits and risks of platform trust and safety efforts, the current state-of-the-art of the field, and where it’s going. Series: "Public Policy and Society" [Public Affairs] [Science] [Show ID: 39285]…
U
UC Berkeley (Video)

1 Debunking Disinformation: Fighting the Fake News Battle with Joan Donovan 28:39
28:39
הפעל מאוחר יותר
הפעל מאוחר יותר
רשימות
לייק
אהבתי28:39
Joan Donovan, a leading disinformation researcher specializing in media manipulation, explains how social media platforms have become the new battleground for public persuasion. Co-author of “Meme Wars: The Untold Story of the Online Battles Upending Democracy in America,” Donovan uncovers the ways memes and social media enable fringe groups to lure in new recruits and spread their ideologies. In this episode, Donovan provides expert guidance on technical and policy strategies necessary to mitigate the weaponization of social media. Series: "Public Policy and Society" [Public Affairs] [Science] [Show ID: 39286]…
U
UC Berkeley (Video)

1 Debunking AI: Ensuring Artificial Intelligence Doesn’t Destroy Our World 24:24
24:24
הפעל מאוחר יותר
הפעל מאוחר יותר
רשימות
לייק
אהבתי24:24
TecHype is a groundbreaking series that cuts through the hype around emerging technologies. Each episode debunks misunderstandings around emerging tech, provides insight into benefits and risks, and identifies technical and policy strategies to harness the benefits while mitigating the risks. This episode of TecHype features Prof. Stuart Russell from UC Berkeley, a world-renowned expert in artificial intelligence and co-author (with Peter Norvig) of the standard text in the field. We debunk misunderstandings around what “AI” actually is and break down the benefits and risks of this transformative technology. Prof. Russell provides an expert perspective on the real impacts AI will have in our world, including its potential to provide greater efficiency and effectiveness in a variety of domains and the serious safety, security, and discrimination risks it poses. Series: "Public Policy and Society" [Public Affairs] [Science] [Show ID: 39284]…
UC Berkeley engineers have created a simple and low-cost new arsenic treatment system to help low-income communities access safer water. In many areas throughout California, the groundwater is tainted with dangerous levels of arsenic, a highly carcinogenic element that can seep into the water table from deposits in the soil and bedrock. While cities and larger municipalities can afford to remove arsenic from their water, many people living in small and rural communities are forced to choose between drinking contaminated tap water or purchasing bottled water — and those with private wells may not even know that their water is unsafe. (Video: Roxanne Makasdjian, Alan Toth, Adam Lau) Series: "UC Berkeley News" [Health and Medicine] [Show ID: 39226]…
Researchers deployed a fleet of 100 semi-autonomous vehicles to test whether a new AI-powered cruise control system can help smooth the flow of traffic and improve fuel economy. In a massive traffic experiment, scientists tested whether introducing just a few AI-equipped vehicles to the road can help ease “phantom” jams caused by human behavior and reduce fuel consumption for everyone. (Video: Roxanne Makasdjian, Alan Toth, and CIRCLES Consortium Music: Dyalla - Organic Guitar House) Series: "UC Berkeley News" [Public Affairs] [Science] [Show ID: 39225]…
U
UC Berkeley (Video)

1 Debunking Deepfakes: Unmasking Digital Deceptions with Hany Farid 28:42
28:42
הפעל מאוחר יותר
הפעל מאוחר יותר
רשימות
לייק
אהבתי28:42
TecHype is a groundbreaking series that cuts through the hype around emerging technologies to get to what matters. Each episode debunks misunderstandings around emerging tech, provides insight into benefits and risks, and identifies technical and policy strategies to harness the benefits while mitigating the risks of emerging technologies. This episode of TecHype features Prof. Hany Farid from UC Berkeley, a world-renowned expert in the analysis of digitally manipulated images. We take a deep dive into determining what a 'deepfake’ is and explore how these AI-generated images, videos, and audio can be used for both amusing and alarming purposes. Farid highlights the increasing prevalence of deepfakes and their impact on society. From revolutionizing the entertainment industry, bolstering creativity, and championing advocacy campaigns to their use in impersonating public figures in ways that manipulate elections or personal contacts to commit fraud. The episode concludes with a discussion of targeted strategies that can be pursued to keep you safe from harmful deepfakes, such as digital watermarking and detection tools. Series: "Public Policy and Society" [Public Affairs] [Science] [Show ID: 39244]…
U
UC Berkeley (Video)

UC Berkeley drills a 400-foot borehole to explore geothermal heating on campus. UC Berkeley plans to decommission its 40-year-old cogeneration plant and replace its current steam heating system with a new system that uses water pipes to heat and cool buildings on campus. While the cogeneration plant burns natural gas to produce electricity and steam heat for the campus, the new system will use electricity for both power and thermal needs. By using clean energy sources, such as wind and solar, to produce this electricity, the campus’s future power, heating and cooling needs would be entirely carbon-free. (Video: Roxanne Makasdjian, Alan Toth, Adam Lau) Series: "UC Berkeley News" [Public Affairs] [Science] [Show ID: 39224]…
U
UC Berkeley (Video)

1 Exploring Communities: Humans and Non-Humans Together 1:55:17
1:55:17
הפעל מאוחר יותר
הפעל מאוחר יותר
רשימות
לייק
אהבתי1:55:17
Using real-life examples and historical evidence, French anthropologist Philippe Descola aims to understand the unique characteristics of communities that exist outside of modern societies. These communities have often been misunderstood because they were mistakenly compared to nation-states. However, Descola argues that we should examine the components and relationships within these communities based on how they perceive the world. By doing so, we can challenge the Eurocentric and human-centric view of social structures, which rely on Western ideas of progress and functionality. Series: "Tanner Lectures on Human Values" [Humanities] [Show ID: 38617]…
U
UC Berkeley (Video)

1 Exploring Modern Communities 1:50:11
1:50:11
הפעל מאוחר יותר
הפעל מאוחר יותר
רשימות
לייק
אהבתי1:50:11
In this program, scholars Philippe Descola, Adom Getachew, Timothy LeCain and David Wengrow discuss how views of humans verses non-humans shaped the modern world. Series: "Tanner Lectures on Human Values" [Humanities] [Show ID: 38618]
U
UC Berkeley (Video)

This series on artificial intelligence explores recent breakthroughs of AI, its broader societal implications and its future potential. In this presentation, Pamela Samuelson, professor of Law and Information at UC Berkeley, discusses whether computer-generated texts and images fall under the copyright law. She says that early on, the consensus was that AI was just a tool, like a camera, so humans could claim copyright in machine-generated outputs to which they made contributions. Now the consensus is that AI-generated texts and images are not copyrightable for the lack of a human author. The urgent questions today focus on whether ingesting in-copyright works as training data is copyright infringement and whether the outputs of AI programs are infringing derivative works of the ingested images. Four recent lawsuits, one involving GitHub’s Copilot and three involving Stable Diffusion, will address these issues. Samuelson has been a member of the UC Berkeley School of Law faculty since 1996. She has written and spoken extensively about the challenges that new information technologies pose for traditional legal regimes, especially for intellectual property law. She is a member of the American Academy of Arts & Sciences, a fellow of the Association for Computing Machinery (ACM), a contributing editor of Communications of the ACM, a past fellow of the John D. & Catherine T. MacArthur Foundation, a member of the American Law Institute, and an honorary professor of the University of Amsterdam. Series: "Data Science Channel" [Science] [Business] [Show ID: 38859]…
U
UC Berkeley (Video)

1 How to Create AI to Solve Real-World Problems 46:29
46:29
הפעל מאוחר יותר
הפעל מאוחר יותר
רשימות
לייק
אהבתי46:29
This series on artificial intelligence explores recent breakthroughs of AI, its broader societal implications and its future potential. In this presentation, Sergey Levine, associate professor of Electrical Engineering and Computer Science at UC Berkeley, discusses AI reinforcement learning methods. Levine asks what it would take to create machine learning systems that can make decisions when faced with the full complexity and diversity of the real world, while still retaining the ability of reinforcement learning to come up with new solutions? He discusses how advances in offline reinforcement learning can enable machine learning systems to learn to make more optimal decisions from data, combining the best of data-driven machine learning with the capacity for emergent behavior and optimization provided by reinforcement learning. Levine received a BS and MS in Computer Science from Stanford University in 2009, and a Ph.D. in Computer Science from Stanford University in 2014. He joined the faculty of the Department of Electrical Engineering and Computer Sciences at UC Berkeley in fall 2016. His work focuses on machine learning for decision making and control, with an emphasis on deep learning and reinforcement learning algorithms. Applications of his work include autonomous robots and vehicles, as well as applications in other decision-making domains. His research includes developing algorithms for end-to-end training of deep neural network policies that combine perception and control, scalable algorithms for inverse reinforcement learning, deep reinforcement learning algorithms, and more. Series: "Data Science Channel" [Science] [Show ID: 38857]…
U
UC Berkeley (Video)

1 How Not To Destroy The World With AI 58:11
58:11
הפעל מאוחר יותר
הפעל מאוחר יותר
רשימות
לייק
אהבתי58:11
This series on artificial intelligence explores recent breakthroughs of AI, its broader societal implications and its future potential. In this presentation, Stuart Russell, professor of computer science at the UC, Berkeley, discusses what AI is and how it could be beneficial to civilization. Russell is a leading researcher in artificial intelligence and the author, with Peter Norvig, of “Artificial Intelligence: A Modern Approach,” the standard text in the field. His latest book, “Human Compatible,” addresses the long-term impact of AI on humanity. He is also an honorary fellow of Wadham College at the University of Oxford. Series: "Data Science Channel" [Science] [Show ID: 38856]…
U
UC Berkeley (Video)

1 How AI Fails Us and How Economics Can Help 50:32
50:32
הפעל מאוחר יותר
הפעל מאוחר יותר
רשימות
לייק
אהבתי50:32
This series on artificial intelligence explores recent breakthroughs of AI, its broader societal implications and its future potential. In this presentation, Michael Jordan, professor of Electrical Engineering and Computer Science and Statistics at UC Berkeley, discusses the how to connect research in economics with computer science and statistics, with a long-term goal of providing a broader conceptual foundation for emerging real-world AI systems, and to upend received wisdom in the computational, economic and inferential disciplines. Jordan argues that AI has focused on a paradigm in which intelligence inheres in a single agent, and in which agents should be autonomous so they can exhibit intelligence independent of human intelligence. Thus, when AI systems are deployed in social contexts, the overall design is often naive. Such a paradigm need not be dominant. In a broader framing, agents are active and cooperative, and they wish to obtain value from participation in learning-based systems. Agents may supply data and resources to the system, only if it is in their interest. Critically, intelligence inheres as much in the system as it does in individual agents. Jordan's research interests bridge the computational, statistical, cognitive, biological and social sciences. He is a member of the National Academy of Sciences, the National Academy of Engineering, and the American Academy of Arts and Sciences, and a foreign member of the Royal Society. He was a plenary lecturer at the International Congress of Mathematicians in 2018. He received the Ulf Grenander Prize from the American Mathematical Society in 2021, the IEEE John von Neumann Medal in 2020, the IJCAI Research Excellence Award in 2016, the David E. Rumelhart Prize from the Cognitive Science Society in 2015 and the ACM/AAAI Allen Newell Award in 2009. Series: "Data Science Channel" [Science] [Business] [Show ID: 38858]…
U
UC Berkeley (Video)

1 A Critical Look At Modern Eurocentric Anthropology 1:55:18
1:55:18
הפעל מאוחר יותר
הפעל מאוחר יותר
רשימות
לייק
אהבתי1:55:18
French anthropologist Philippe Descola examines the evolution of modern thinking about societies. He argues that the rooting of the descriptive tools of the social sciences in Enlightenment philosophy has blinded us to the fact that what are loosely called ‘societies’ are in fact, for extra-moderns, assemblages that, unlike ours, contain and associate much more than just humans, either because their institutions are able to integrate other-than-humans into collectives, or because other-than-humans are seen as political subjects acting within their own collectives. In other words, the kinds of beings that result from these assemblages are not those to which philosophy or the social sciences usually pay attention: they are associations of humans and other-than-humans that take very diverse forms and, in this sense, can also offer food for thought about the much-needed transformation of the political and social institutions proper to the Moderns. We could call these assemblages cosmopolities in that they bring under the same regime of cosmic sociability a vast set of components that the ontology of the Moderns has tended to dissociate. Series: "Tanner Lectures on Human Values" [Humanities] [Show ID: 38616]…
U
UC Berkeley (Video)

1 How Modern Slavery Touches Everyone 1:23:41
1:23:41
הפעל מאוחר יותר
הפעל מאוחר יותר
רשימות
לייק
אהבתי1:23:41
Modern slavery, which encompasses 45 million people around the world, is intricately linked to the economy, politics, violence and war, gender and the environment. In this panel discussion, Kevin Bales, professor of contemporary slavery and research director of the Rights Lab at the University of Nottingham, talks about the impact of contemporary slavery with three UC Berkeley professors, Arlie Hochschild, professor emerita, Department of Sociology, Enrique Lopezlira, Ph.D., director, Low-Wage Work Program, and Eric Stover, adjunct law professor and faculty director, Human Rights Center. Slave-based activities, like brick making and deforestation, are estimated to generate 2.54 billion tonnes of CO2 per year – greater than the individual emissions of all the world’s nations except China and the U.S. Globally, slaves are forced to do work that is highly destructive to the environment. This work feeds directly into global consumption in foodstuffs, in minerals – both precious and for electronics – construction materials, clothing, and foodstuffs. Most of this work is unregulated leading to extensive poisoning of watersheds, the clear-cutting of forests, and enormous and unregulated emissions of carcinogenic gases as well as CO2. Political corruption supports this slave-based environmental destruction and its human damage. Series: "UC Berkeley Graduate Lectures" [Humanities] [Science] [Business] [Show ID: 38615]…
U
UC Berkeley (Video)

1 How Modern Slavery Impacts the Environment with Kevin Bales 1:27:12
1:27:12
הפעל מאוחר יותר
הפעל מאוחר יותר
רשימות
לייק
אהבתי1:27:12
There are 45 million enslaved people in the world today. The links between slavery, conflict, environmental destruction, economics and consumption began to strengthen and evolve in the 20th century. The availability of people who might be enslaved dramatically increased in line with population growth. According to Kevin Bales, professor of contemporary slavery and research director of the Rights Lab at the University of Nottingham, the large and negative environmental impact of modern slavery is just now coming to light. Slave-based activities, like brick making and deforestation, are estimated to generate 2.54 billion tonnes of CO2 per year – greater than the individual emissions of all the world’s nations except China and the U.S. Globally, slaves are forced to do work that is highly destructive to the environment. This work feeds directly into global consumption in foodstuffs, in minerals – both precious and for electronics – construction materials, clothing, and foodstuffs. Most of this work is unregulated leading to extensive poisoning of watersheds, the clear-cutting of forests, and enormous and unregulated emissions of carcinogenic gases as well as CO2. Political corruption supports this slave-based environmental destruction and its human damage. Kevin Bales, CMG, FRSA is Professor of Contemporary Slavery and Research Director of the Rights Lab, University of Nottingham. He co-founded the American NGO Free the Slaves. His 1999 book Disposable People: New Slavery in the Global Economy has been published in twelve languages. Desmond Tutu called it “a well researched, scholarly and deeply disturbing expose of modern slavery.” The film based on Disposable People, which he co-wrote, won the Peabody Award and two Emmys. The Association of British Universities named his work one of “100 World-Changing Discoveries.” In 2007 he published Ending Slavery: How We Free Today’s Slaves (Grawemeyer Award). In 2009, with Ron Soodalter, he published The Slave Next Door: Modern Slavery in the United States. In 2016 his research institute was awarded the Queens Anniversary Prize, and he published Blood and Earth: Modern Slavery, Ecocide, and the Secret to Saving the World. Check out his TEDTalk. Series: "UC Berkeley Graduate Lectures" [Humanities] [Science] [Business] [Show ID: 38614]…
U
UC Berkeley (Video)

1 Constructing a Republican Executive with Michael McConnell 1:20:13
1:20:13
הפעל מאוחר יותר
הפעל מאוחר יותר
רשימות
לייק
אהבתי1:20:13
As the delegates to the Constitutional Convention gathered in Philadelphia in 1789, there was no experience, anywhere in the world, of a successful republican executive over an extensive nation — one with sufficient authority and independence to make things work on a national scale, but without the risk of becoming a monarch. This Jefferson Memorial Lecture shows how the delegates, and especially the Committee of Detail, went about constructing such an executive, and what it means for separation-of-powers law today. Series: "UC Berkeley Graduate Lectures" [Public Affairs] [Show ID: 38613]…
U
UC Berkeley (Video)

1 For the People? Representative Government in America 1:58:50
1:58:50
הפעל מאוחר יותר
הפעל מאוחר יותר
רשימות
לייק
אהבתי1:58:50
It has become commonplace that democracy in the United States faces an existential threat. This belief has gained popular currency in the wake of Donald Trump’s presidency, nourished by his conduct in office, the attempt to overturn the 2020 election, and continuing efforts to subvert the electoral process. Whether this is true only time will tell. But a common narrative among scholars of American government holds that representative democracy is failing more systematically than the Trump phenomenon suggests. In this program, Charles Beitz, professor of politics at Princeton University, along with commentary by Martin Gilens, Pamela S. Karlan and Jane Mansbridge, talk about the current state of democratic dysfunction and what the future might hold. Series: "Tanner Lectures on Human Values" [Public Affairs] [Show ID: 38274]…
U
UC Berkeley (Video)

1 For the People? Representative Government in America: Regulating Rivalry 1:58:58
1:58:58
הפעל מאוחר יותר
הפעל מאוחר יותר
רשימות
לייק
אהבתי1:58:58
It has become commonplace that democracy in the United States faces an existential threat. This belief has gained popular currency in the wake of Donald Trump’s presidency, nourished by his conduct in office, the attempt to overturn the 2020 election, and continuing efforts to subvert the electoral process. Whether this is true only time will tell. But a common narrative among scholars of American government holds that representative democracy is failing more systematically than the Trump phenomenon suggests. In this program, Charles Beitz, professor of politics at Princeton University, along with commentary by Pamela S. Karlan and Jane Mansbridge, talk about how to regulate rivalry in democratic representative government. Series: "Tanner Lectures on Human Values" [Public Affairs] [Show ID: 38273]…
U
UC Berkeley (Video)

1 For the People? Representative Government in America: Intimations of Failure 1:52:40
1:52:40
הפעל מאוחר יותר
הפעל מאוחר יותר
רשימות
לייק
אהבתי1:52:40
It has become commonplace that democracy in the United States faces an existential threat. This belief has gained popular currency in the wake of Donald Trump’s presidency, nourished by his conduct in office, the attempt to overturn the 2020 election, and continuing efforts to subvert the electoral process. Whether this is true only time will tell. But a common narrative among scholars of American government holds that representative democracy is failing more systematically than the Trump phenomenon suggests. In this program, Charles Beitz, professor of politics at Princeton University, and Martin Gilens, professor of public policy at UCLA, address how to diagnose the problem of whether or not our system of government is failing. Series: "Tanner Lectures on Human Values" [Public Affairs] [Show ID: 38272]…
U
UC Berkeley (Video)

1 21st Century Global Health Priorities with Christopher Murray 1:27:03
1:27:03
הפעל מאוחר יותר
הפעל מאוחר יותר
רשימות
לייק
אהבתי1:27:03
The world has lived through 2+ years of the COVID-19 pandemic, heightening the awareness of the links between health and other aspects of life including education and the economy. Future pandemics are a real risk but there are a number of other threats to human health and well-being as well. These include climate change, the rise of obesity, inverted population pyramids, inter-state conflict, rising inequalities, antimicrobial resistance. Counterbalancing these threats are the opportunities that may come through the health sector and broader innovation. Using a comprehensive future health scenario framework, Christopher Murray, professor and chair of Health Metrics Sciences, University of Washington and director of the Institute for Health Metrics and Evaluation, explores the range of future trajectories that may unfold in the 21st century. Series: "UC Berkeley Graduate Lectures" [Public Affairs] [Health and Medicine] [Business] [Show ID: 38271]…
U
UC Berkeley (Video)

1 The Social Safety Net as an Investment in Children with Hilary Hoynes 1:17:45
1:17:45
הפעל מאוחר יותר
הפעל מאוחר יותר
רשימות
לייק
אהבתי1:17:45
A hallmark of every developed nation is the provision of a social safety net – a collection of public programs that deliver aid to the poor. Because of their higher rates of poverty, children are often a major beneficiary of safety net programs. Compared to other countries, the U.S. spends less on antipoverty programs and, consequently, has higher child poverty rates. Professor Hilary Hoynes discusses the emerging research that examines how the social safety net affects children’s life trajectories. The long run benefits are significant for the families, but also show that many programs prove to be excellent public investments. This has implications for current policy discussions such as the expanded Child Tax Credit. Series: "UC Berkeley Graduate Lectures" [Public Affairs] [Business] [Show ID: 38275]…
U
UC Berkeley (Video)

1 The Status Quo Loves To Say No: Disability Rights and the Battle Against Structures of Exclusion with Judith Heumann 1:28:31
1:28:31
הפעל מאוחר יותר
הפעל מאוחר יותר
רשימות
לייק
אהבתי1:28:31
Disability rights activist Judy Heumann has been fighting for inclusion for over six decades, in ways that transformed legal and societal understandings of equality. Her life-long experience has included co-founding the organization Disabled in Action, working on Capitol Hill to shape landmark disability rights laws, co-organizing the extraordinary protest and advocacy efforts that spurred the implementation of Section 504, and advising presidential administrations and the World Bank on disability issues. In this conversational program, Heumann focuses on those aspects of her journey that are most salient to the perils and possibilities of the present. Heumann sees in this moment a fragile and imperfect democracy, but one that is nonetheless worth investing in. She also sees deep structures of exclusion, vigorously defended but also vulnerable to political pressure and moral suasion. Series: "UC Berkeley Graduate Lectures" [Health and Medicine] [Humanities] [Show ID: 37730]…
U
UC Berkeley (Video)

1 Metrics in Action: Lessons Learned from 30 Years of the Global Burden of Disease Study with Christopher Murray 1:15:28
1:15:28
הפעל מאוחר יותר
הפעל מאוחר יותר
רשימות
לייק
אהבתי1:15:28
The Global Burden of Disease Study (GBD) began in 1991 sponsored by the World Bank and the World Health Organization to fill a critical gap in global health information. It has grown steadily to become an active collaboration of more than 8,000 scientists, researchers and policy-makers from 156 countries working together to quantify health at the national and subnational level. In this program, Christopher J.L. Murray, Professor and Chair of Health Metrics Sciences at the University of Washington and Director of the Institute for Health Metrics and Evaluation (IHME), discusses what we've learned over the last 30 years studying global health. Series: "UC Berkeley Graduate Lectures" [Public Affairs] [Health and Medicine] [Show ID: 38270]…
U
UC Berkeley (Video)

Ukrainian Member of Parliament Inna Sovsun joins Yuriy Gorodnichenko, Professor of Economics at UC Berkeley, and Janet Napolitano, Professor at the Goldman School of Public Policy and former Secretary of Homeland Security, for a firsthand perspective on the war in Ukraine since the Russian invasion in early 2022. They discuss the war's impact, and what comes next for the people of Ukraine. This event is cosponsored by the Goldman School of Public Policy, the Center for Security in Politics, the Center for Studies in Higher Education, the Institute of Slavic, East European, and Eurasian Studies, the Institute of European Studies Series: "The Goldman School - Berkeley Public Policy" [Public Affairs] [Show ID: 38606]…
U
UC Berkeley (Video)

1 Center for Security in Politics Fireside Chat on US-Mexico Relations 1:29:32
1:29:32
הפעל מאוחר יותר
הפעל מאוחר יותר
רשימות
לייק
אהבתי1:29:32
UC Berkeley Goldman School's new Center for Security in Politics hosts a fireside chat with former UC President and Secretary of Homeland Security Janet Napolitano in conversation with Jose Antonio Meade. He served as a cabinet minister under Presidents Felipe Calderón and Enrique Peña Nieto in a variety of roles, including Secretary of Finance and Public Credit, Secretary of Social Development, Secretary of Foreign Affairs, and Secretary of Energy. He was the Institutional Revolutionary Party (PRI) candidate in the 2018 presidential election, where he placed third. Napolitano is a Professor of Public Policy and Director of the new Center for Security in Politics. An in-depth conversation about U.S.-Mexico relations, the challenges of the border, and how we can align forces to build a better future for both countries. Series: "The Goldman School - Berkeley Public Policy" [Public Affairs] [Show ID: 38556]…
U
UC Berkeley (Video)

1 The Demarcation Problem for Philosophy 1:55:42
1:55:42
הפעל מאוחר יותר
הפעל מאוחר יותר
רשימות
לייק
אהבתי1:55:42
Philosophy almost alone among disciplines appears to lack a distinctive subject matter. The world has chemical, biological and political aspects, but no philosophical aspects. If subject matter does have a role to play here, it’s to do less with the field’s descriptive ambitions than the genealogy of philosophical problems. MIT's professor of philosophy's Stephen Yablo’s interests are wide-ranging, from metaphysics, philosophical logic and epistemology, to the philosophy of language and the philosophy of mathematics. A prolific writer, Yablo is the author of a two-volume collection of papers called Thoughts: Papers on Mind, Meaning, and Modality (2009) and Things: Papers on Objects, Events, and Properties (2010). His most recent book, Aboutness (2014), attempts to make topic an equal partner in meaning with truth-conditions, applying meanings so conceived to knowledge, logic, ontology, discourse theory, and philosophical methodology. Series: "UC Berkeley Graduate Lectures" [Humanities] [Show ID: 37732]…
U
UC Berkeley (Video)

1 Smart Money: Educational Investments in Adolescents Earn Higher Returns 1:57:29
1:57:29
הפעל מאוחר יותר
הפעל מאוחר יותר
רשימות
לייק
אהבתי1:57:29
This program looks at education and how the brain of adolescents primes them for learning. Caroline Hoxby, professor of economics at Stanford University and one of the world’s leading scholars in the field of education economics, says investing in education in early adolescents can have profound impacts on the continuation of learning through teenage years and beyond. She says that more education leads to social cohesion, advancing economic opportunity, reducing inequality and decreasing geographic and political polarization. This discussion includes commentary by Jan-Werner Müller, Erik Hurst, and Silvia Bunge. Series: "Tanner Lectures on Human Values" [Public Affairs] [Education] [Show ID: 37733]…
U
UC Berkeley (Video)

1 The Fork in the Road: Investing in Adolescent Education 1:47:34
1:47:34
הפעל מאוחר יותר
הפעל מאוחר יותר
רשימות
לייק
אהבתי1:47:34
One of the world’s leading scholars in the field of education economics, Caroline Hoxby’s lectures draw upon economics, neuroscience, and education. She shows that early adolescence is the point at which most people either join the path towards advanced cognitive skills or not. Hoxby argues that it is imperative to invest in early education. Series: "Tanner Lectures on Human Values" [Public Affairs] [Education] [Show ID: 38061]…
U
UC Berkeley (Video)

1 The Fork in the Road: Adolescence Education Economic Fatalism and Populism with Caroline Hoxby 1:49:39
1:49:39
הפעל מאוחר יותר
הפעל מאוחר יותר
רשימות
לייק
אהבתי1:49:39
One of the world’s leading scholars in the field of education economics, Caroline Hoxby’s lectures draw upon economics, neuroscience, and education. She shows that early adolescence is the point at which most people either join the path towards advanced cognitive skills or not. Hoxby argues that, in a highly industrialized economy like that of the United States, it is not unreasonable for people on the non-advanced-cognitive-skills path to exhibit “economic fatalism” — despair at their long-term economic prospects. This may explain patterns in persons’ susceptibility to populism and political movements that promise to insulate them from the world economy. Series: "Tanner Lectures on Human Values" [Public Affairs] [Education] [Show ID: 37731]…
U
UC Berkeley (Video)

1 A Discussion on Artificial Intelligence with Kate Crawford 1:17:45
1:17:45
הפעל מאוחר יותר
הפעל מאוחר יותר
רשימות
לייק
אהבתי1:17:45
Kate Crawford, author of "Atlas AI: Power, Politics and Planetary Costs of Artificial Intelligence," is a leading international scholar of the social and political implications of artificial intelligence. This program looks at the intersection of art, activism and artificial intelligence. Series: "Tanner Lectures on Human Values" [Humanities] [Science] [Show ID: 37967]…
U
UC Berkeley (Video)

1 Excavating Ground Truth in AI: Epistemologies and Politics in Training Data with Kate Crawford 56:57
56:57
הפעל מאוחר יותר
הפעל מאוחר יותר
רשימות
לייק
אהבתי56:57
The last decade has seen a dramatic capture of digital material for machine learning production. This data is the basis for sense-making in AI, not as classical representations of the world with individual meaning, but as mass collections: ground truth for machine abstractions and operations. What happens when data is seen as an aggregate, stripped of context, meaning, and specificity? In what ways does training data limit what and how machine learning systems interpret the world? And most importantly, what forms of power do these approaches enhance and enable? Professor Kate Crawford is a leading international scholar of the social and political implications of artificial intelligence. In this lecture, Crawford shares new work that reflects on what’s at stake in the architecture and contents of training sets, and how they are increasingly part of our urban, legal, logistical, and commercial infrastructures. Series: "Tanner Lectures on Human Values" [Humanities] [Science] [Show ID: 37729]…
U
UC Berkeley (Video)

1 A New Measure: The Revolutionary Quantum Reform of the Modern Metric System 1:27:19
1:27:19
הפעל מאוחר יותר
הפעל מאוחר יותר
רשימות
לייק
אהבתי1:27:19
The International System of Units (the SI), the modern metric system, has recently undergone its most revolutionary change since its origins during the French Revolution. The nature of this revolution is that all of the base units of the SI are now defined by fixing values of natural constants. Our measurement system is now, both philosophically and practically, strongly quantum. Nobel Prize recipient William Phillips, Ph.D., a Distinguished University and College Park Professor of Physics at the University of Maryland, talks about why this reform was needed and how it is done. Series: "UC Berkeley Graduate Lectures" [Science] [Show ID: 37728]…
Tilden Park’s Jewel Lake is home to a community of Western pond turtles, a species that is struggling to survive the combined threats of climate change, habitat loss and competition from red-eared slider turtles. Former UC Berkeley postdoctoral scholar Max Lambert is part of a team of wildlife experts who spent much of the pandemic checking in on the health of the Bay Area’s Western pond turtles, including those at Jewel Lake. Series: "UC Berkeley News" [Agriculture] [Show ID: 37841]…
U
UC Berkeley (Video)

1 Time Einstein and the Coolest Stuff in the Universe 1:28:50
1:28:50
הפעל מאוחר יותר
הפעל מאוחר יותר
רשימות
לייק
אהבתי1:28:50
At the beginning of the 20th century, Einstein changed the way we think about time. Now, early in the 21st century, the measurement of time is being revolutionized by the ability to cool a gas of atoms to temperatures millions of times lower than any naturally occurring temperature in the universe. Nobel Prize recipient William Phillips, Ph.D., a Distinguished University and College Park Professor of Physics at the University of Maryland, talks about laser cooling and ultracold atoms and how they relate to time. Atomic clocks, the best timekeepers ever made, are one of the scientific and technological wonders of modern life. Such super-accurate clocks are essential to industry, commerce, and science; they are the heart of the Global Positioning System (GPS), which guides cars, airplanes, and hikers to their destinations. Today, the best primary atomic clocks use ultracold atoms. Series: "UC Berkeley Graduate Lectures" [Science] [Show ID: 37727]…
UC Berkeley’s Kroeber Hall became the fourth building on campus to be stripped of its name in a year’s time. The decision by Berkeley officials capped a formal review process and was made, in large part, because the building’s namesake - Alfred Louis Kroeber, born in 1876 and the founder of the study of anthropology in the American West - is a powerful symbol that continues to evoke exclusion and erasure for Native Americans. Series: "UC Berkeley News" [Humanities] [Show ID: 37827]…
ברוכים הבאים אל Player FM!
Player FM סורק את האינטרנט עבור פודקאסטים באיכות גבוהה בשבילכם כדי שתהנו מהם כרגע. זה יישום הפודקאסט הטוב ביותר והוא עובד על אנדרואיד, iPhone ואינטרנט. הירשמו לסנכרון מנויים במכשירים שונים.