התחל במצב לא מקוון עם האפליקציה Player FM !
פודקאסטים ששווה להאזין
בחסות


1 You Can Visit All Seven Continents. But Should You? 26:46
Transformer Memory as a Differentiable Search Index: memorizing thousands of random doc ids works!?
Manage episode 355037188 series 3446693
Andrew Yates and Sergi Castella discuss the paper titled "Transformer Memory as a Differentiable Search Index" by Yi Tay et al at Google. This work proposes a new approach to document retrieval in which document ids are memorized by a transformer during training (or "indexing") and for retrieval, a query is fed to the model, which then generates autoregressively relevant doc ids for that query.
Paper: https://arxiv.org/abs/2202.06991
Timestamps:
00:00 Intro: Transformer memory as a Differentiable Search Index (DSI)
01:15 The gist of the paper, motivation
4:20 Related work: Autoregressive Entity Linking
7:38 What is an index? Conventional vs. "differentiable"
10:20 Indexing and Retrieval definitions in the context of the DSI
12:40 Learning representations for documents
17:20 How to represent document ids: atomic, string, semantically relevant
22:00 Zero-shot vs. finetuned settings
24:10 Datasets and baselines
27:08 Dinetuned results
36:40 Zero-shot results
43:50 Ablation results
47:15 Where could this model be useds?
52:00 Is memory efficiency a fundamental problem of this approach?
55:14 What about semantically relevant doc ids?
60:30 Closing remarks
Contact: castella@zeta-alpha.com
21 פרקים
Manage episode 355037188 series 3446693
Andrew Yates and Sergi Castella discuss the paper titled "Transformer Memory as a Differentiable Search Index" by Yi Tay et al at Google. This work proposes a new approach to document retrieval in which document ids are memorized by a transformer during training (or "indexing") and for retrieval, a query is fed to the model, which then generates autoregressively relevant doc ids for that query.
Paper: https://arxiv.org/abs/2202.06991
Timestamps:
00:00 Intro: Transformer memory as a Differentiable Search Index (DSI)
01:15 The gist of the paper, motivation
4:20 Related work: Autoregressive Entity Linking
7:38 What is an index? Conventional vs. "differentiable"
10:20 Indexing and Retrieval definitions in the context of the DSI
12:40 Learning representations for documents
17:20 How to represent document ids: atomic, string, semantically relevant
22:00 Zero-shot vs. finetuned settings
24:10 Datasets and baselines
27:08 Dinetuned results
36:40 Zero-shot results
43:50 Ablation results
47:15 Where could this model be useds?
52:00 Is memory efficiency a fundamental problem of this approach?
55:14 What about semantically relevant doc ids?
60:30 Closing remarks
Contact: castella@zeta-alpha.com
21 פרקים
כל הפרקים
×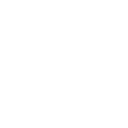
1 AGI vs ASI: The future of AI-supported decision making with Louis Rosenberg 54:42
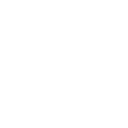
1 EXAONE 3.0: An Expert AI for Everyone (with Hyeongu Yun) 24:57
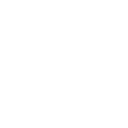
1 Zeta-Alpha-E5-Mistral: Finetuning LLMs for Retrieval (with Arthur Câmara) 19:35
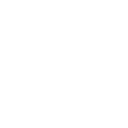
1 ColPali: Document Retrieval with Vision-Language Models only (with Manuel Faysse) 34:48
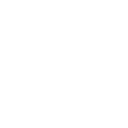
1 Using LLMs in Information Retrieval (w/ Ronak Pradeep) 22:15
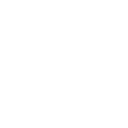
1 Designing Reliable AI Systems with DSPy (w/ Omar Khattab) 59:57
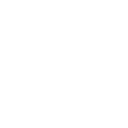
1 The Power of Noise (w/ Florin Cuconasu) 11:45
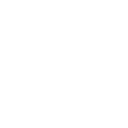
1 Benchmarking IR Models (w/ Nandan Thakur) 21:55
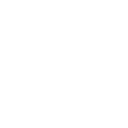
1 Baking the Future of Information Retrieval Models 27:05
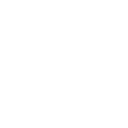
1 Hacking JIT Assembly to Build Exascale AI Infrastructure 38:04
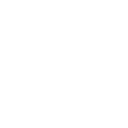
1 The Promise of Language Models for Search: Generative Information Retrieval 1:07:31
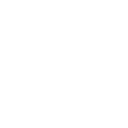
1 Task-aware Retrieval with Instructions 1:11:13
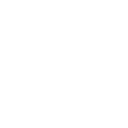
1 Generating Training Data with Large Language Models w/ Special Guest Marzieh Fadaee 1:16:14
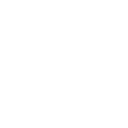
1 ColBERT + ColBERTv2: late interaction at a reasonable inference cost 57:30
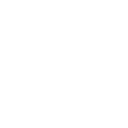
1 Evaluating Extrapolation Performance of Dense Retrieval: How does DR compare to cross encoders when it comes to generalization? 58:30

1 Open Pre-Trained Transformer Language Models (OPT): What does it take to train GPT-3? 47:12

1 Few-Shot Conversational Dense Retrieval (ConvDR) w/ special guest Antonios Krasakis 1:23:11

1 Transformer Memory as a Differentiable Search Index: memorizing thousands of random doc ids works!? 1:01:40

1 Learning to Retrieve Passages without Supervision: finally unsupervised Neural IR? 59:10

1 The Curse of Dense Low-Dimensional Information Retrieval for Large Index Sizes 54:13

1 Shallow Pooling for Sparse Labels: the shortcomings of MS MARCO 1:07:17
ברוכים הבאים אל Player FM!
Player FM סורק את האינטרנט עבור פודקאסטים באיכות גבוהה בשבילכם כדי שתהנו מהם כרגע. זה יישום הפודקאסט הטוב ביותר והוא עובד על אנדרואיד, iPhone ואינטרנט. הירשמו לסנכרון מנויים במכשירים שונים.