32 subscribers
התחל במצב לא מקוון עם האפליקציה Player FM !
פודקאסטים ששווה להאזין
בחסות


1 #52: Navigating the effect of AI on marketing jobs and the job market with Sue Keith, Landrum Talent Solutions 19:09
Build a Real Time AI Data Platform with Apache Kafka
Manage episode 424666729 series 2510642
Is it possible to build a real-time data platform without using stateful stream processing? Forecasty.ai is an artificial intelligence platform for forecasting commodity prices, imparting insights into the future valuations of raw materials for users. Nearly all AI models are batch-trained once, but precious commodities are linked to ever-fluctuating global financial markets, which require real-time insights. In this episode, Ralph Debusmann (CTO, Forecasty.ai) shares their journey of migrating from a batch machine learning platform to a real-time event streaming system with Apache Kafka® and delves into their approach to making the transition frictionless.
Ralph explains that Forecasty.ai was initially built on top of batch processing, however, updating the models with batch-data syncs was costly and environmentally taxing. There was also the question of scalability—progressing from 60 commodities on offer to their eventual plan of over 200 commodities. Ralph observed that most real-time systems are non-batch, streaming-based real-time data platforms with stateful stream processing, using Kafka Streams, Apache Flink®, or even Apache Samza. However, stateful stream processing involves resources, such as teams of stream processing specialists to solve the task.
With the existing team, Ralph decided to build a real-time data platform without using any sort of stateful stream processing. They strictly keep to the out-of-the-box components, such as Kafka topics, Kafka Producer API, Kafka Consumer API, and other Kafka connectors, along with a real-time database to process data streams and implement the necessary joins inside the database.
Additionally, Ralph shares the tool he built to handle historical data, kash.py—a Kafka shell based on Python; discusses issues the platform needed to overcome for success, and how they can make the migration from batch processing to stream processing painless for the data science team.
EPISODE LINKS
- Kafka Streams 101 course
- The Difference Engine for Unlocking the Kafka Black Box
- GitHub repo: kash.py
- Watch the video version of this podcast
- Kris Jenkins’ Twitter
- Streaming Audio Playlist
- Join the Confluent Community
- Learn more with Kafka tutorials, resources, and guides at Confluent Developer
- Live demo: Intro to Event-Driven Microservices with Confluent
- Use PODCAST100 to get an additional $100 of free Confluent Cloud usage (details)
פרקים
1. Intro (00:00:00)
2. What is Forecasty.ai? (00:01:43)
3. Using AI techniques for forecast modeling (00:03:20)
4. Migrating from batch to real-time stream processing (00:09:51)
5. Getting started with Apache Kafka (00:13:08)
6. Building kash.py—a Python-based Kafka shell (00:23:52)
7. Future plans for using Kafka (00:31:10)
8. It's a wrap! (00:35:44)
265 פרקים
Manage episode 424666729 series 2510642
Is it possible to build a real-time data platform without using stateful stream processing? Forecasty.ai is an artificial intelligence platform for forecasting commodity prices, imparting insights into the future valuations of raw materials for users. Nearly all AI models are batch-trained once, but precious commodities are linked to ever-fluctuating global financial markets, which require real-time insights. In this episode, Ralph Debusmann (CTO, Forecasty.ai) shares their journey of migrating from a batch machine learning platform to a real-time event streaming system with Apache Kafka® and delves into their approach to making the transition frictionless.
Ralph explains that Forecasty.ai was initially built on top of batch processing, however, updating the models with batch-data syncs was costly and environmentally taxing. There was also the question of scalability—progressing from 60 commodities on offer to their eventual plan of over 200 commodities. Ralph observed that most real-time systems are non-batch, streaming-based real-time data platforms with stateful stream processing, using Kafka Streams, Apache Flink®, or even Apache Samza. However, stateful stream processing involves resources, such as teams of stream processing specialists to solve the task.
With the existing team, Ralph decided to build a real-time data platform without using any sort of stateful stream processing. They strictly keep to the out-of-the-box components, such as Kafka topics, Kafka Producer API, Kafka Consumer API, and other Kafka connectors, along with a real-time database to process data streams and implement the necessary joins inside the database.
Additionally, Ralph shares the tool he built to handle historical data, kash.py—a Kafka shell based on Python; discusses issues the platform needed to overcome for success, and how they can make the migration from batch processing to stream processing painless for the data science team.
EPISODE LINKS
- Kafka Streams 101 course
- The Difference Engine for Unlocking the Kafka Black Box
- GitHub repo: kash.py
- Watch the video version of this podcast
- Kris Jenkins’ Twitter
- Streaming Audio Playlist
- Join the Confluent Community
- Learn more with Kafka tutorials, resources, and guides at Confluent Developer
- Live demo: Intro to Event-Driven Microservices with Confluent
- Use PODCAST100 to get an additional $100 of free Confluent Cloud usage (details)
פרקים
1. Intro (00:00:00)
2. What is Forecasty.ai? (00:01:43)
3. Using AI techniques for forecast modeling (00:03:20)
4. Migrating from batch to real-time stream processing (00:09:51)
5. Getting started with Apache Kafka (00:13:08)
6. Building kash.py—a Python-based Kafka shell (00:23:52)
7. Future plans for using Kafka (00:31:10)
8. It's a wrap! (00:35:44)
265 פרקים
كل الحلقات
×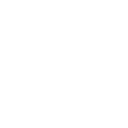
1 Apache Kafka 3.5 - Kafka Core, Connect, Streams, & Client Updates 11:25
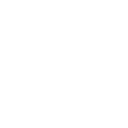
1 How to use Data Contracts for Long-Term Schema Management 57:28
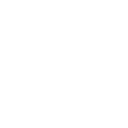
1 How to use Python with Apache Kafka 31:57
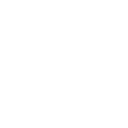
1 Next-Gen Data Modeling, Integrity, and Governance with YODA 55:55
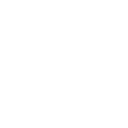
1 Migrate Your Kafka Cluster with Minimal Downtime 1:01:30
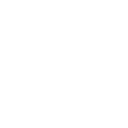
1 Real-Time Data Transformation and Analytics with dbt Labs 43:41
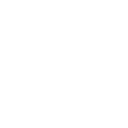
1 What is the Future of Streaming Data? 41:29
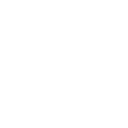
1 What can Apache Kafka Developers learn from Online Gaming? 55:32
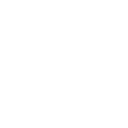
1 How to use OpenTelemetry to Trace and Monitor Apache Kafka Systems 50:01
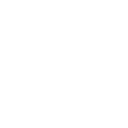
1 What is Data Democratization and Why is it Important? 47:27
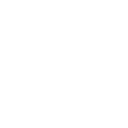
1 Git for Data: Managing Data like Code with lakeFS 30:42
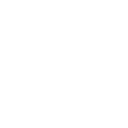
1 Using Kafka-Leader-Election to Improve Scalability and Performance 51:06
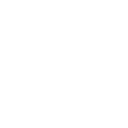
1 Real-Time Machine Learning and Smarter AI with Data Streaming 38:56

1 The Present and Future of Stream Processing 31:19

1 Top 6 Worst Apache Kafka JIRA Bugs 1:10:58

1 Learn How Stream-Processing Works The Simplest Way Possible 31:29

1 Building and Designing Events and Event Streams with Apache Kafka 53:06

1 Rethinking Apache Kafka Security and Account Management 41:23

1 Real-time Threat Detection Using Machine Learning and Apache Kafka 29:18

1 Improving Apache Kafka Scalability and Elasticity with Tiered Storage 29:32

1 Decoupling with Event-Driven Architecture 38:38

1 If Streaming Is the Answer, Why Are We Still Doing Batch? 43:58

1 Security for Real-Time Data Stream Processing with Confluent Cloud 48:33

1 Running Apache Kafka in Production 58:44

1 Build a Real Time AI Data Platform with Apache Kafka 37:18

1 Optimizing Apache JVMs for Apache Kafka 1:11:42


1 Application Data Streaming with Apache Kafka and Swim 39:10

1 International Podcast Day - Apache Kafka Edition | Streaming Audio Special 1:02:22


1 Real-Time Stream Processing, Monitoring, and Analytics With Apache Kafka 34:07

1 Reddit Sentiment Analysis with Apache Kafka-Based Microservices 35:23

1 Capacity Planning Your Apache Kafka Cluster 1:01:54

1 Streaming Real-Time Sporting Analytics for World Table Tennis 34:29

1 Real-Time Event Distribution with Data Mesh 48:59

1 Apache Kafka Security Best Practices 39:10

1 What Could Go Wrong with a Kafka JDBC Connector? 41:10

1 Apache Kafka Networking with Confluent Cloud 37:22

1 Event-Driven Systems and Agile Operations 53:22

1 Streaming Analytics and Real-Time Signal Processing with Apache Kafka 1:06:33

1 Blockchain Data Integration with Apache Kafka 50:59

1 Automating Multi-Cloud Apache Kafka Cluster Rollouts 48:29

1 Common Apache Kafka Mistakes to Avoid 1:09:43

1 Building Real-Time Data Governance at Scale with Apache Kafka ft. Tushar Thole 42:58

1 Handling 2 Million Apache Kafka Messages Per Second at Honeycomb 41:36


1 Serverless Stream Processing with Apache Kafka ft. Bill Bejeck 42:23

1 The Evolution of Apache Kafka: From In-House Infrastructure to Managed Cloud Service ft. Jay Kreps 46:32


1 Intro to Event Sourcing with Apache Kafka ft. Anna McDonald 30:14

1 Expanding Apache Kafka Multi-Tenancy for Cloud-Native Systems ft. Anna Povzner and Anastasia Vela 31:01


1 Optimizing Cloud-Native Apache Kafka Performance ft. Alok Nikhil and Adithya Chandra 30:40

1 From Batch to Real-Time: Tips for Streaming Data Pipelines with Apache Kafka ft. Danica Fine 29:50

1 Real-Time Change Data Capture and Data Integration with Apache Kafka and Qlik 34:51

1 Modernizing Banking Architectures with Apache Kafka ft. Fotios Filacouris 34:59

1 Running Hundreds of Stream Processing Applications with Apache Kafka at Wise 31:08

1 Lessons Learned From Designing Serverless Apache Kafka ft. Prachetaa Raghavan 28:20

1 Using Apache Kafka as Cloud-Native Data System ft. Gwen Shapira 33:57

1 ksqlDB Fundamentals: How Apache Kafka, SQL, and ksqlDB Work Together ft. Simon Aubury 30:42

1 Explaining Stream Processing and Apache Kafka ft. Eugene Meidinger 29:28

1 Handling Message Errors and Dead Letter Queues in Apache Kafka ft. Jason Bell 37:41

1 Confluent Platform 7.0: New Features + Updates 12:16

1 Real-Time Stream Processing with Kafka Streams ft. Bill Bejeck 35:32

1 Automating Infrastructure as Code with Apache Kafka and Confluent ft. Rosemary Wang 30:08

1 Getting Started with Spring for Apache Kafka ft. Viktor Gamov 32:44

1 Powering Event-Driven Architectures on Microsoft Azure with Confluent 38:42

1 Automating DevOps for Apache Kafka and Confluent ft. Pere Urbón-Bayes 26:08

1 Intro to Kafka Connect: Core Components and Architecture ft. Robin Moffatt 31:18

1 Designing a Cluster Rollout Management System for Apache Kafka ft. Twesha Modi 30:08

1 Apache Kafka 3.0 - Improving KRaft and an Overview of New Features 15:17

1 How to Build a Strong Developer Community with Global Engagement ft. Robin Moffatt and Ale Murray 35:18
ברוכים הבאים אל Player FM!
Player FM סורק את האינטרנט עבור פודקאסטים באיכות גבוהה בשבילכם כדי שתהנו מהם כרגע. זה יישום הפודקאסט הטוב ביותר והוא עובד על אנדרואיד, iPhone ואינטרנט. הירשמו לסנכרון מנויים במכשירים שונים.